Welcome to LearnLawBetter, where today we are going to discuss the story behind
Pennoyer v. Neff, a case that most law students read in their civil procedure casebook.
In addition to the story, stay to the end for the rule of law from the case.
Hi, this is Beau Baez, and today I want to talk to you about Pennoyer v. Neff, a case
I read during the first year of law school and which is still required reading in many
Civil Procedure courses.
The Supreme Court case revolves around three people: Marcus Neff, the plaintiff; Sylvester
Pennoyer, the defendant; and the main figure in our story today, John Hipple Mitchell—
a U.S. Senator when the case was decided.
Mitchell was born with the name John Mitchell Hipple in Pennsylvania.
He became a school teacher, and was forced to marry a 15 year old student who he got
pregnant.
He then became a lawyer, but decided he was unhappy in Pennsylvania so in 1860
he left his wife, stole today's equivalent of $112,000 in client funds, and left for
California with his mistress.
When his mistress got sick, Hipple abandoned her in California, moved up to Oregon, and
there changed his last name from Hipple to Mitchell.
Neff needed legal help in securing land that he was trying to get under the Federal Donation
Act, a federal law to encourage people to move to Oregon so Neff went to Mitchell for legal advice.
Before Neff received legal title to the land he had settled, he left Oregon, at which point
Mitchell filed suit for unpaid legal bills.
Under Oregon law, Mitchell was required to provide notice by publication if the defendant
could not be found in Oregon.
Now apparently Oregon law did not specify the type of publication, so Mitchell placed the
notice in a Methodist publication called the Pacific Christian Advocate—I mean almost certainly
a ploy to make sure that Neff would never find out about the law suit against him.
Of course, Neff did not show up for trial so Mitchell won the case in early 1866, but
Mitchell held on to the judgment for a few months until he received notice that the federal
government had in fact given legal title to Neff for the land that he had settled.
Now this fact is important, as Neff did not legally own the property in Oregon until after the trial
was over.
Mitchell then purchased the land at auction, and a few days later assigned the land to
Pennoyer, a person who eventually was to become a governor of the State of Oregon.
Now let's Fast forward eight years, when in 1874 Neff suddenly shows up and claims that the land was still
his, claiming that the Oregon courts did not have jurisdiction over him at the time of
the original lawsuit.
By this time Mitchell was one of Oregon's two U.S. Senators, though he was not a named
party in the lawsuit.
The case made it's way up all the way to the U.S. Supreme Court, which held Oregon did not have jurisdiction
over Neff under the Due Process Clause of the 14th Amendment.
Now I always find it interesting when people talk about the good old days, when people were more moral
than they are today.
Frankly, there is nothing new under the sun.
When Mitchell became a U.S. Senator, it was revealed that he had never divorced his first
wife back in Pennsylvania, which meant that he was a bigamist.
Just before Mitchell's second election to the Senate, the newspapers published a series of letters
proving that Mitchell had a five year affair with his current wife's sister—Mitchell,
nonetheless, won reelection.
In 1905, Senator Mitchell was convicted of federal land fraud and sentenced to 6 months
in prison.
Before the Senate could expel him, he died from complications related to some dental
work.
You'll be glad to know that since he died a Senator, the Senate did vote to cover his
funeral expenses.
I'd like to send a shout out to Dean Wendy Perdue at the University of Richmond School of Law,
who taught me Pennoyer v. Neff when I was a law student at Georgetown.
Now for the rule of law: a court has jurisdiction to hear a lawsuit involving an out-of-state
resident when the state court has personal jurisdiction over the out-of-state resident;
or, when the out-of-state resident owns property in the state.
Neff won this case because he was not in Oregon at the time the lawsuit began, and he did
not have legal title to the property at the time of the lawsuit—he received legal title
from the federal government after Mitchell's legal billing lawsuit.
If you liked this episode hit the like button.
Also, let me know in the comments section how my videos are helping you, and tell me
what topics you want me to cover in a future episode.
We have many other episodes, so feel free to explore.
Also, at LearnLawBetter.com you will find more free resources to help you succeed, including
our newsletter, blog posts, and exam bank.
Thank you for watching.
For more infomation >> Sex & the Senator in Pennoyer v. Neff - Duration: 5:17.-------------------------------------------
The Thinkquisition is Here - Duration: 6:52.
So Last Friday, the progressive website Mother Jones included me in an article about a quote
"new extremist media industry that is further to the right than Breitbart."
In the piece, the author referred to me as "far right", citing interviews I've done with
Mike Cernovich, Milo Yiannopoulos and Lauren Southern to back up his accusation.
He cherry picked these guests to fit his narrative, yet somehow missing the endless amount of
liberals I've had on and the progressives I've hosted, including Margaret Cho, Hillary
Rosen, Mark Duplass, John Fugelsang and Michael Ian Black.
The article also attacked Patreon, the crowdfunding platform of which myself and my team's livelihood
depends on, for being tolerant of people with far right views such as mine, though of course,
nowhere did it include any of my purported "far right" views.
Earlier in the week, Forbes called The Rubin Report a "conservative talk show", but immediately
retracted the statement when I contacted them.
Ironically, I've been saying for awhile defending my liberal beliefs is becoming a conservative
position.
Although I don't think being called a conservative is somehow bad, it clearly isn't a proper
descriptor for this show in which I've hosted people from across the political divide.
For Mother Jones to accuse me of being an extremist, further to the right of Breitbart,
is obviously an inflammatory and demonstrably dishonest statement.
I've been around many people who over the last few years have been unfairly maligned,
and I'm all to familiar with how this all goes.
Whether it's Sam Harris, Jordan Petersen, Colin Moriarty or Brett Weinstein -- each
attack is virtually never based on what these people have said, but are instead crafted
out of straw man arguments with the words racist and bigot tossed around like candy.
Suddenly, the onus is on people who aren't racists or bigots to disprove this accusation,
usually slung by a misguided celebrity, or a malicious newspaper or website.
The move, then, is on the accused -- should they spend time and energy defending themselves
from accusations which aren't true, only to bring more attention to the accusations themselves?
Or should they ignore ignore them, hoping the false claims will go away over time.
I think there are solid arguments to be made for either tactic, and it truly depends on
the situation at hand and the person involved.
In this case, though, I went on the attack.
I decided this case was too egregious to ignore, contacting the article's author via Twitter,
screenshotting pieces of his article and asking him to defend them.
Of course, he couldn't defend his own words...that's the thing with truth, it's hard to work around...and
eventually he made slight changes to the article, like including a tweet of mine in which I
defended myself from his claims, and he removing the word "far" in front of the word "right"
before referencing me in the article.
Putting aside the specifics of this article itself, I was happy to get a strong defense
in public from many friends all over the political map.
People like Joe Rogan, Colin Moriarty, Faisal Saeed al Mutar, Bret Weinstein, Eric Weinstein,
Michael Shermer, Peter Boghossian and many others offered strong defenses of me, just
as I've offered for others when I've seen them unfairly attacked.
I even got a public defense from Donald Trump Jr.
Putting my friends and public allies aside though, it's incredibly inspiring and humbling
to see literally 10's of thousands of people offer their voice when they feel you've been
unjustly attacked.
And that's the key to this whole thing.
It's not me, or Sam, or Jordan or Bret that they fear, what they really fear is that all
of you will starting saying what you think ... because There's strength in numbers.
By Monday, I wanted to put the whole story behind me.
My dog has a health emergency, which helped put everything in perspective, but I admit,
I did get sucked back into the attack very briefly.
Over the course of a Twitter exchange, I got the writer of the piece, Josh Harkinson, to
publicly admit I am NOT further right than Breitbart, though of course, he didn't change
the title or have Mother Jones issue any sort of public correction.
One other interesting piece to all this, is that the writer, John Harkinson, is one of
the 40 people that James Hodgkinson, the shooter of the Republican baseball team last week,
followed on Twitter.
Now I can't draw a direct link there, but we know that Hodgkinson was a far lefty who
obviously was driven to political extremes.
So, when this very same writer does a hatchet job on me calling me further right than Breitbart,
which is clearly code for racist, I cannot sit back there and take it.
This is exactly why I've been saying you can't punch Nazi's.
Call everyone you oppose a Nazi and a racist and then eventually you will see violence
as the next logical step.
It's not a crazy exercise to think that this is partially what drove James Hodgkinson.
In hindsight his attack on me was the next obvious step in where this whole collectivist
movement is going.
I've talked for a long time about how if you can't debate ideas, you use lies and buzzwords.
Having it turned on me shouldn't have been a surprise, especially from a Leftist site
like Mother Jones, yet it still did surprise me somehow.
Make no mistake, there is a Thinkquisition going on here, and it can come for anyone
at any time.
If you ally yourself with people who value the movement, in this case a political one,
over you as an individual, then eventually it is inevitable that they will come for you.
And if they don't come for you, you're probably the one coming for them.
This Thinkquisition will come after anyone who bucks the trend and the stereotypes and
dares think for themselves.
It's why they come accuse Ayaan Hirsi Ali of being anti muslim, it's why they slander
Bill Maher as a being racist, it's why they call Larry Elder an uncle tom, and it's why
I can be branded a far right extremist.
Those who are a part of this Thinkquisition fear you thinking for yourself, that you won't
bow to their collectivist demands, more than anything else.
The way to beat this witch hunt is to be yourself, and fight for what you believe.
You are the antidote they fear most.
I hope that next time you see me fighting back and getting some cover from my allies,
it will inspire you to fight back... specifically with words, not violence... as well.
Joining me on the show this week is one of my aforementioned allies and friends, author
and professor Peter Boghossian, who will help me dissect the madness of 2017 and much more.
-------------------------------------------
Dr. Jekyll & Mr. Hyde – Thug Notes Summary & Analysis - Duration: 8:23.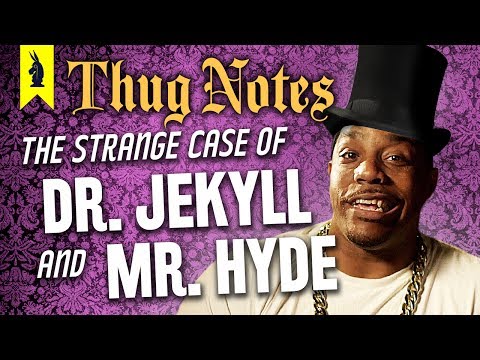
What's good y'all? This week, we doublin' down wit The Strange Case of Dr. Jekyll and
Mr. Hyde, by Robert Louis Stevenson. Lawyer-man John Utterson swangin' down da streets of
London when his cous Enfield start givin him the DL on a CRAZY-a** story. Brutha like:
"Yo- Back in da winter I peeped this shady cat named Hyde straight STOMP a b**** JUST
CUZ she bumped into him. Soon as I saw dat mess, I told him he best throw a C-note her
way or else me and this mob gonna turn this into a scandal." Hyde like "Psh.
fine" and oblige a brutha, but dat check is signed by an uptown gangsta with solid
rep, which Enfield thinkin' is hella strange.
Soon Utterson recognize that da name on the check is a client of his named Dr. Jekyll.
So after grubbin at da crib, Utterson check his files and learn dat this Jekyll cat is
leavin all his cheddar to Mr. Hyde. Hm. Dat don't sound right. Utterson decide to drop
in on one of Jekyll's homeboys, Dr. Lanyon. But Lanyon say he ain't seein Dr. J's
crazy a** since they had a beef about his bulls*** research ten years ago. Utterson
start scopin' out a spot dat Hyde been seen at. Boy creepin up on da house one night when
Hyde rolls by. They conversate fo' a sec befo' Hyde ducks into a lab behind Jekyll's
pad. After a dinner party up at Jekyll's, Utterson ask him what the deal is with Mr.
Hyde. But Dr. J just shakes that load off and say "don't trip bout dat, baby."
Nearly a year later, Utterson get word that somebody in town got iced, so he pay a visit
to the po-lice. Turns out, Mr. Hyde dun ghosted one of his old crusty clients. Utterson leads
the fuzz to Hyde's traphouse, but dude ain't nowhere to be found. Utterson hit up Dr. J
to get some answers, but Jekyll say "I don't f*** with that dude no mo. He long gone."
and hands him a note from Hyde sayin he's sorry for all his bulls***. Thang is, though,
both Dr. Jekyll and Mr. Hyde got da same handwriting. Hmmm! Fo the next two months Doctor J back
to his regular self, but dat don't last long. Soon he don't let anyone come to his
crib no mo, and next thing you know- his bro Dr. Lanyon drops dead. Lanyon leave Utterson
a letter. But he ain't s'posed to open it til something raw happen to Jekyll. What?!
S*** gets real when Jekyll's butler tell Utterson that Doc J dun locked his self in
dat lab fo MONTHS! And the voice on the other side of the door sho as HELL ain't Jekyll's.
Maybe he been merced! So the two of em' break the door down, an' find Mr. Hyde dead
in Jekyll's clothes. From da look of it, dude killed hisself by sippin on some poison
juice. Utterson find an updated will, a note tellin him to read lanyon's letter, and
a confession from Hyde that gonna explain errything. Utterson head home to read Lanyon's
letter, which say he started losin his damn mind when he saw Mr. Hyde turn into Dr. Jekyll.
Da shock ate at his mind til it eventually put him in the ground. Da second letter sayin
how Dr. J been turning himslf into a nappy monster that bring out da dark side of his
personality. Dat way, he can do cray s*** on the reg without no consequences. Doc Jekyll
was havin a good ol time morphin into dat gnarly beast til he started transforming in
his SLEEP. Knowin this s*** too cray to keep in line, the doc kicked da habit for a while,
but when he startin jonezin fo dem dark deeds, he gave in, turned in to Hyde, and merced
dat old geezer.
After a while, Jekyll lose so much control dat he start morphin' into Hyde durin'
the DAY. So he hit up Dr. Lanyon fo some syrup to change him back. Sadly, Jekyll just kept
transformin'. Eventually brutha couldn' find da legit goods to make the amount of
serum to keep Hyde in check. Knowin dat he gonna be Mr. Hyde fo'eva soon, he decide
to off his-self. His letter end by sayin he got a new will leavin' Utterson all his
cheddar. So this ending ain't ALL bad!
Any literary OG'll tell you Jekyll an' Hyde
is a primo example of gothic lit. It's packin' everythang you'd expect: night scenes, s****y
weather, viscious deaths, TRANSFO'MATIONS, a creepy house wit scurry secrets, an' a
freaky lab wit more dark corners than a porno theater. God DAMN! But da darkest thing goin
down in this novella ain't even dat- it's what Stevenson sayin' bout human nature.
See back in his day, scientists thought dat one half of yo brain reppin a certain kinda
personality and desires, and da other half reppin da exact opposite.
Righteous balla Jekyll was smart, chill an civilized 'cause that's what doctors thought
da left side of the brain was like. Whereas Mr. Hyde is always comin' out HARD gettin
all savage n' s*** cuz homies thought the right half was mo' primal and impulsive.
Stevenson slangin images of animals to associate Hyde with dat beastly side. This nappy scrub
growls like a dog and throws his limbs around like a damn monkey! Perhaps it ain't even
dat he look like an animal, but just dat Mr. Hyde's soul is so evil, people just THINK
he a fiend!
Or maybe he s'posed to represent da OG beastie: dat hater-snake from the Garden of Eden. When
he morphs back into Jekyll in front of Dr. Lanyon, he talk in hisses, an' even try
to f*** wit Lanyon's head by appealing to his desire for dank-a** knowledge:
Dat boy might as well be the devil tempting Eve to grub on dat apple from the tree of
knowledge! But Lanyon ain't the only dude to see a lil Hell in Hyde. Jekyll talk bout
his other half as "that child of hell," and Utterson says he see "satan's signature"
in Hyde's face. Dayum! Hyde's mug is so janky, it's like he's straight up bible-level
evil. Da real question we all gotz to ask ourselves is: Are we all on da same struggle
as po' Dr. Jekyll? Are we all one drink away from becoming MONSTERS?
Maybe Stevenson's jam is a warning about livin' in denial. No one can keep
their dark side hidden forever. So you should be be true to yo'self and recognize da good
AND bad parts. Only then can a brutha truly find balance. You feel me?
Yo, thanks for checkin out Thug Notes. Peace yall!
-------------------------------------------
Things That Make Me Happy - Spring 2017 | @laurenfairwx - Duration: 6:51.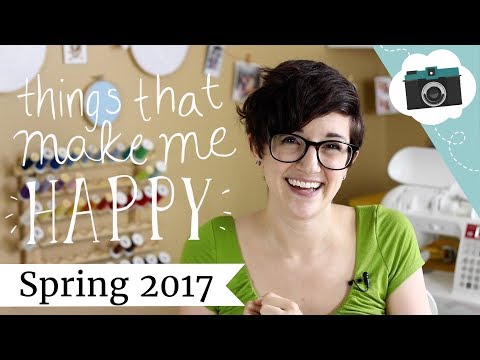
Just under the wire, I wanted to do a spring edition of my Things That Make Me
Happy series. I think of this as a more conceptual kind of favorites video
because some of my choices are pretty general. But honestly, the things that you
don't have to go out and buy are sometimes just as happy-making as the
products that I really like. I might as well just get started, so here is another
list of things that make me happy! First up for this episode: Easter candy. The
best time of year for chocolate, in my opinion. Russell Stover milk chocolate
covered marshmallows and the Cadbury caramel eggs are some of my absolute
favorites. But for some reason the Reese's Peanut Butter Cup eggs taste so
much better to me than just normal Reese's peanut butter cups that you can
get all year long. I don't know why that is. Maybe because it's fresher and it can
only sit on the shelf for a limited amount of time before it becomes
outdated and it goes to clearance, which, clearance Easter candy is also something
that makes me really happy. After Easter is over, they mark it all down and it's
still just as delicious, so I just go and buy a whole bunch of it and then try to
make it last as long as I can. I know it's a silly thing, but I get so excited
for Easter time because, I just, the chocolate makes me really happy.
I don't even wait until Easter to eat it. I start eating it after Valentine's Day
when it appears in the stores magically and it's just the best thing. The next
thing on my list is long walks. It sounds so simple but after being
cooped up all winter long, trying to walk in the snow and people just don't shovel
their sidewalks in my neighborhood, it is so nice to go outside, sometimes without
a coat, and just enjoy the fresh air and I love it. There are nice things to look
at, I sometimes run into friends in my neighborhood and get to catch up, and it
makes me feel a lot better. If I feel like I've been cooped up all day and
just like sitting and working for hours, it's so nice to go out for a walk. It
inspires me to do work, so when I come home, I have like a ton of new ideas to
work on. It's something that I've found is really good for my mental health. I
love it. I love going for walks! The next thing that makes me happy is Julie's
Greenroom. This is Julie Andrews' Netflix original show and it
just is so cute! I was so excited when I heard that the show was going to happen
because it's like everything that I wanted in a show when I was a kid. I have
a one and a half year old. Getting to enjoy it with him, and I'm sure I'm going
to watch it again when he's a little bit older and can really understand all of the
concepts, but it's a show about theater and the arts! It's Julie Andrews teaching
a new generation of kids about musicals and it's so positive and sweet. There's a
really great special guest on every episode. I really needed this show right
now. It's just, it makes me really really happy and I highly recommend it if you
haven't watched it, especially if you're a theatre person. So the next item on my
list is Japanese stationery. Ever since I got into traveler's notebooks, I've been
looking online at all sorts of cute stickers and pens and I feel like I've
been gravitating the most towards things that come from Japan. I've been a little
bit overwhelmed with all of the options, so I haven't bought very much Japanese
stationery, but then Zenpop, which is a stationary subscription service located
in Japan, offered to send me a box of their stuff so I said yes. Knowing me, you
may be able to imagine the restraint and the patience that it required for me to
not open this until I got on camera, but I'm going to check it out now. Oh it's
really cute. There's actually a lot of stuff in here!
Stickers and a little memo pad, oh my goodness this is cute.
I don't know what it is about this stuff. It like, makes me really excited. Some
really adorable pencils. Oh my gosh, washitape with cats on it. Little sushi
stickers! Anyway, that gave me a really good place to start with my Japanese
stationery addiction, so thank you, Zenpop. I think I'm in trouble
because I'm going to go buy a whole bunch more now. The next thing is Roller
Coaster Tycoon Classic for the iPad. Mobile games are great, I really like
them, I play a whole lot of them, but any time I play any mobile game that's at
all theme park related, I keep thinking back to when I was in middle school and
I would play Rollercoaster Tycoon, the original version of it, and I kept
thinking, how do all these mobile games that have all this new technology at
their disposal, not coming even close to at least what I remembered of the
Rollercoaster Tycoon of my childhood? So the people who made Rollercoaster Tycoon
decided to re-release the original version of
Rollercoaster Tycoon but reformatted for the iPad. I was so excited when I found
this out. It really is just as good as I remember, I don't know if the children of
today would feel the same way, because I'm sure it's a nostalgia element. I
think it is so much fun to play. It's such a silly, fun game but it's a huge
game! It's massive! There are so many parks. It includes some of the original
expansion packs as well. If you ever played that game as a child, you have to
look it up. I cannot recommend it highly enough. And the last thing on my list of
things that make me happy for spring, is Instagram! Now, I've been on Instagram for
a long time. I really enjoy it. It's an extra creative outlet for me and I love
the idea that I can take a photo on my phone and then mess with it in a bunch
of different apps for a while, and then post it and share it with people
immediately and I just think that's really fun! Another thing that I love
about Instagram is that it is what you make it, so if you want to follow a ton
of artists that inspire you, all you have to do is open up Instagram and just
scroll through your feed and when I do that I get inspired, I get excited to
make things again. It sometimes can turn my whole mood around and just make my
day a little bit happier and that's kind of amazing. People are always quick to be
negative about social media and there are a lot of negative things about it.
But I find that Instagram is one you can use it in this specific way, to only
follow things that make you smile and that can be kind of powerful, you know?
I've also been posting more often on Instagram lately because it's been
making me so happy, so if you want to follow me on Instagram and see what I've
been posting, I've got a whole bunch of behind the scenes stuff for my channel
and my crafts and my shows that I perform at! Look me up on Instagram at
@laurenfairwx. Okay! So that's it for now. What kinds of things have been
making you happy lately? Tell me in a comment below and hopefully I'll have a
chance to reply if I can. I love chatting with all of you in the comments. If
you're new here and you liked this, don't forget to subscribe and hit the
notification bell so you don't miss any of my future videos. I've been working on
a lot of happy and creative stuff lately and I can't wait to share it with you.
Thank you for watching and I'll see you soon!
-------------------------------------------
Corsa-Spec Alfa Romeo GTA Sparks Its Pilot's Passion - Duration: 8:56.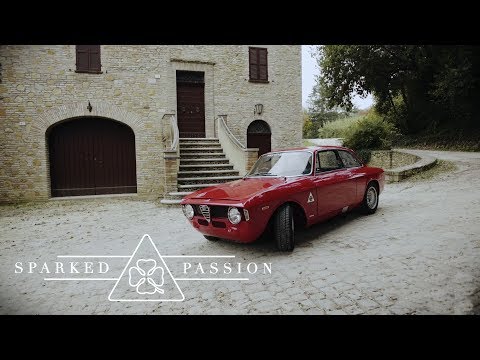
The passion I have for this car is not due to
its value
or because it is rare,
nor for its heritage.
It's just that when I get in it, and I close the door,
I am in my own special world.
I tune everything out.
I leave for a timeless dimension,
it is where I go to blow off steam.
This car gives me emotions that cannot be put into words.
It is such an intimate experience,
that I jealously cherish it.
My name is Filippo Montini
I was born in 1976,
and my passion has always been
for 1960's Alfa Romeos.
I have owned this Alfa Romeo Giulia GTA for 10 years.
GTA stands for Gran Turismo Alleggerita.
It's not my family's first car because
my love for these cars started a long time ago.
My grandfather was a test driver for Benelli Moto,
my father always worked on cars as a hobby
and as a trade.
So racing was a big part of my childhood,
both for motorcylces and cars.
When I was 8 years old, in an aviation field,
I saw a Giulia.
I was star-strucked by that car,
and from that moment on I felt something
that I cannot put into words.
It was very peculiar because
we met the owner of the Giulia, Angelo,
and he immediately seemed trustworthy.
A friendship was born that day that lasts to today.
Me and my dad were supervising the restoration of a car
that was particularly complexed.
[Angelo], the owner of the GTA, came from Brescia
to Pesaro, where we were,
Just to meet us and we had lunch together.
We talked about my passion for the cars I owned,
the ones I wanted and the ones that Angelo had.
Angelo told me he had a disassembled GTA for the last 10 years,
because he did not have any time to fix it himself.
So my dad suggested that he could put it together.
So we bought the GTA sight un-seen because it was disassembled,
without thinking what the build would entail.
Without assessing the risks
that we could run into with a car of this type.
We went to see the car,
we saw that Angelo had been truthful,
so we started to divide all the pieces,
and organize them in different crates.
We took all the crates home, and little by little like a puzzle,
we were able to re-build the whole car.
Obviously it was a job that took 3 years.
But it paid off because we got the certificate of authenticity
from both the "Registro Italiano Alfa Romeo" and
and from Maurizio Tabucchi,
a famous GTA expert who recently passed away.
The main characteristics in a GTA
is it's aluminum riveted body.
This is to keep the weight of the car low.
You have a 200 kilos lighter car than the most common
aluminum version.
The car is also lighter thanks to alloy wheels,
and the engine is more powerful thanks to the dual ignition system.
What is commonly known as "twin sparks",
with 2 sparks for each cilinder.
The engine with a street configuration has 115 horse power.
But my car has 160 horse power because
it has a racing configuration.
It is also right-hand driven,
it is part of circa 49 cars built in Udine,
at Auto Delta,
that already came out of the factory with more horse powers.
The restoration process lasted around 3 years,
and Angelo, the previous owner, was very influential.
Because he supervised the mechanical part of the build.
So I would see and talk to Angelo often,
just as I still do now, because we remained good friends.
So now when he calls, the first thing he asks me is not how I am
but rather when was the last time that I drove the GTA.
I think he is really happy with this re-built, because
his cars is in good hands,
and even if this car is now mine, a small part will always be his too.
It is a car that will always be with me.
-------------------------------------------
[Japanese ASMR] Marshmallow Eating Sounds #2 / Whispering - Duration: 15:01.
-------------------------------------------
Dota 2 Tricks: Permanent Invisibility during FIGHTS! - Duration: 2:25.
-------------------------------------------
BMW 5 Serie Touring 528I High Executive Aut 8 Navi Prof./Xenon/Pano. Dak/Leder/Sportzetels/PDC V+A/B - Duration: 1:01.
-------------------------------------------
BMW 7 Serie 740Le iPerformance High Executive Night Vision |Soft Close | Massagefunctie v+a | Bowers - Duration: 1:00.
-------------------------------------------
Peugeot 5008 1.6 THP 156 pk ST Premiere 7P. | Navi | Panoramadak | PDC v+a | Bluetooth carkit | ECC - Duration: 0:54.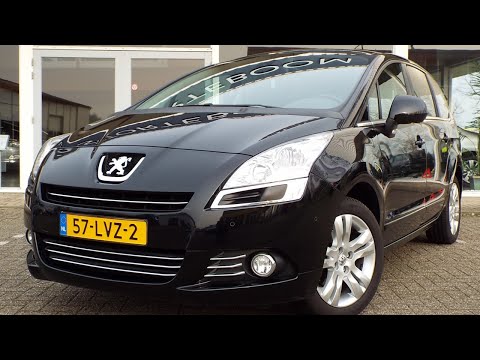
-------------------------------------------
Banzz and Banzz Family Travels to Japan! Osaka, Japan Travel Part 1! - Duration: 19:22.
Off to Osaka!
Ta-da.
We have arrived!
We all slept the whole ride.
(Everyone was tired from staying up the night before)
Hyo-gi will go ahead and guide us now since
he is our translator, a guide, and also our friend.
- Hi, Hyo-gi. - Yes, hello.
- Which way? - We have to go up.
(Going into the city from the airport...)
The clouds are so pretty. So fluffy.
It's not coming out on camera.
(Traveling to Namba by the train!)
Ta-da.
I'm so tired.
Finally at Namba station.
Today, we're first going to drop off our stuff
at a luggage storage.
Then we're going to head to Dotonbori to eat good food and to just relax.
(Dotonbori: Main downtown in Osaka known as the food heaven)
(There are many unique signs and various food places!)
If we go to the left, the street food that we've been looking for will show up.
The street food.
There's so many people out here because it's Sunday.
Hyo-gi.
Is this a lot of people? Or is it the usual amount?
Now? This is the usual amount.
Oh, really?
Not too many or too little?
This is the average.
Is it kinda like Myeongdong in Korea?
There are people are eating right now. It's 3.
That's the Shitenoh Ramen.
Isn't that small octopus?
- What? - Small octopus.
Yeah, I guess a new one opened.
Takoyaki.
That's the Kinryu ramen place we saw.
- Oh, that's Kinryu ramen? - Yeah.
The gold dragon.
A Kushikatsu daruma chain opened here.
This was the guy I was talking about.
Their original one is in Tsutenkaku.
They have good skewers.
And there's another Kanidouraku here.
Let's first try a takoyaki from a place where there isn't a lot of people.
- So our first food here is takoyaki. - Yes.
Takoyaki.
Japan is basically takoyaki..
Takoyaki is Japan.
Right.
Can we order inside?
(Arrived at a less crowded takoyaki place)
Okay. Go Hyo-gi.
(Interesting octopus sign, as expected from Dotonbori)
(Guide Hyo-gi is translating for them)
You guys eat.
(Banzz Family shares takoyaki together)
I was hungry.
Eat this.
(Banzz caring for his younger friends)
Try this one too.
You can have your hands in the shot.
How many hours has it been since we last ate?
12 hours?
I like this one more than the original.
Also...
this is ginger ale.
Ginger.
There's coke too but...
Ginger isn't too common in Korea, right?
Since I'm in Japan...
It's hot. Wow, so ugly.
I think this every time I eat this.
It's something I shouldn't try ever again.
But then I get curious again so I eat it.
There's 4 left.
Let's each eat... there's 4 of us and we bought 8.
We can each eat 2.
Here, you each eat one.
You guys aren't eating because the camera's on.
It's like you're the main event.
Earlier, you guys were surprised when I was ordering.
Was it 4,200 yen. Was it 4,300 yen?
4,200 yen.
How much is that in KRW?
42,000 won.
42,000 won for takoyaki?
There's more to come.
(Takoyaki eaten with soup)
It's so hot. You have to be careful.
The egg gives off a strong taste eating it like this.
I'm able to enjoy the original flavor of the takoyaki more.
You guys eat too.
Jin-hyung, you haven't tried it yet, right?
I'm okay.
Try this one. It's almost the same.
You have to try it at least.
There are plenty of other foods.
We kind of had just an appetizer here.
We'll head off to try other appetizers now.
I'm more hungry now. That was too little.
(Looking for other foods to eat)
Isn't this a famous place?
Let's try one more place.
(Eating more kakoyaki!)
Can you order 10?
- It's 650 yen. - 650?
(It's too hot for Banzz LOL)
You really have to be careful with these.
(His face is red LOL)
Their outer batter is a bit harder.
And the insides are softer.
I think this place is better.
Eat.
It bursts.
Yeah,
The outer batter is crispy 'til the end.
(LOL)
It's seriously so hot. Be careful.
It's hot but Hyo-gi can't make a sound because we're filming.
When...
the harder outside shell bursts, the insides are gooey like the rice cake...
Wow.
This place is better than the last one.
Really?
I'll let this one cool and try it.
Eat this one. It's crispier.
Put the sauce on there and eat this one.
This one's kinda all over the place.
I think you're supposed to eat these right away.
It's crispy on top.
We'll take our time eating these and move to another place after.
We ordered 3 ramens and they're out.
Everything is self-service here.
I'm grabbing spoons and chopsticks.
You can also serve yourself the minced garlic, kimchi, and the scallions.
I'll take this much.
I'm going to try one with and one without.
(Chugging down the food)
Try the one with kimchi.
It's kinda like the janchi noodles.
It ruins the soup.
Right?
It ruins the soup. Adding the kimchi.
Have you tried the one without any toppings?
It just has pepper in it.
I this that one's the best.
It's good to...
have warm soup though.
This one's the best.
We left Kinryu and came this place that sells a variety of things.
It's a yaki (grilled food) place.
Yaki?
The grill is all ready.
I'll go inside.
Let's just end it here.
(Tepanyaki mukbang will be revealed on a Banzz Road video!)
(Heading back to the hotel after eating)
There's a longer line than before.
(Banzz is enjoying the relaxation)
Yeah, it doesn't say 24.
This is just on the streets in Japan?
(Figure shop on the streets!)
Is this what you were talking about before?
This is a different one.
- That's different? - Mazinger Z.
(Japanese convenience store that sells various ingredients)
Honestly, this is too much.
(Plain rice balls?)
Is it just plain rice?
No, it has pickles inside.
Really?
Is it good?
It's called umeboshi...
- Japanese people really like it. - This?
(Buying healthy food even in Japan)
He's buying healthy food to eat even when he's in Japan.
You're curious about Japanese cucumbers?
Japanese tomatoes and cucumbers?
This is 2,000 won?
Isn't that expensive?
Yeah.
(Banzz is surprised how expensive tomatoes are)
This is about 1,800 won too.
Isn't that expensive too?
Tomatoes are too much.
There's isn't much in there either.
I'll just buy this one.
It's basically a cafe here.
(Quality desserts at a convenience store)
Hey.
What is that?
This is only 3,000 won.
What?
What is that?
What is that?
It's the place to be for otakus.
Entertainment... hobbies.
I think they sell figures.
Hey.
This is it.
That's 30,000 yen?
(This place is just minutes from the place we're staying...)
- We'll go inside. - Yeah. Let's.
This is it.
(Decided to head to the hotel first and then come back later)
Us Banzz Family got some rest, organized videos and
did some other few things
and are back outside.
It's close to 1AM now.
We thought we'd check out how the streets are at night.
It's bedtime for us right now originally.
Right? I mean,
it's the time that we're most active.
We'll check out what Japan has to offer in the middle of the night.
Is it the Japan that we expect? Or...
not. We'll check it out.
There's the famous Glico Man sign here.
This is a rare picture, guys.
You would've most likely seen pictures with the neon sign on.
You're seeing the Glico Man sign with the lights off!
It's a rare sight that I'm letting you guys see right now.
This is it.
Over there.
There are street food over there.
It's so pretty to see.
Walking along the river.
Yeah.
And apparently, the Dotonbori that we know of
refers to this river right here.
This whole place is called Dotonbori because this river is so famous.
This river's name is Dotonbori.
It's very dirty, apparently.
Right?
What you guys see over there is the Ferris wheel.
It was a Ferris wheel on top of the Don Quixote.
But after an accident, I heard they no longer operate.
(Is Banzz crying?)
Dong-geun was out here earlier and bought this.
He bought it from Don Quixote.
I heard it's supposed to ease the eyes if you put them in.
At first,
it makes your eyes really sore
so you close you eyes like this but then when you open them,
it feels like you've eaten peppermint candy with your eyes.
It cools your eyes.
Why are you crying?
Mom...
Now we're
inside Don Quixote.
Aren't these doriyaki?
- Doraemon. - Doraemon's favorite.
Doraemon!!
There's a lot of food being sold here
but I plan on doing the shopping later all at once.
So I'm not buying anything yet.
I heard there are many unique drinks sold here.
So here I am.
I can't hold back from drinking what's good~
Anyone that gets a taste goes like this!
Qoo~
I haven't seen these in forever.
Minions.
(Ramune with minions on the cover)
Hyo-gi found them.
You bought them? You didn't pay yet.
- Why did you say you bought them? - I did?
Yeah.
- You're drunk. - I said I bought them?
Dong-geun must have really wanted to try it.
We were supposed to wait until we get to our rooms.
(You have to push the top down with Ramune)
Should we do it on the floor?
(Re-try)
Dong-geun. Go ahead.
Dong-geun. Go ahead and try.
Hyo-gi. Do you wanna try?
Me?
Hit it hard.
I'll use my fist.
Sorry.
(Jin-hyung unexpectedly gets a shower)
So we just drink it like this?
The bead fell inside.
Wow. The bead fell inside.
I think the bead adds the carbonation.
It's all gone now, right?
Yeah, it's all on me.
It's good.
Hey.
There's the bead in here.
The bead just moves around in here.
Yeah. It was stuck up here
and it went down when we hit it.
And I took all the carbonation.
I thought when the bead fell in,
I thought it would melt and
the carbonation would spread.
But it's just a glass bead that falls in.
Dong-geun.
Head to the floor.
That's what you bought?
Famous Ichiran ramen is right next to the Don Quixote.
(Ichiran ramen mukbang will be revealed through Banzz road!)
We'll go ahead and try the Ichiran ramen.
The sun is rising.
It's a long day.
It's getting brighter.
This is what we were originally wanted to do.
All living together and
come up with ideas of our contents and stuff.
Dang it.
We're almost back to our hotel now.
As we were walking back,
the sun came up.
We're using this day to its fullest. Aren't we?
Yeah.
I don't think we'll be able to do anything tomorrow morning.
You mean today morning.
Right. Today morning.
Just moments later.
So sleepy.
End of our Banzz Family's first day!
(See you guys on the next episode!)
(Was that an owl sound?)
(Executive producer : Banzz, Filming: Jo Jin-hyung, Editing Jang Dong-geun)
(Wish I could visit Osaka again)
-------------------------------------------
5 WEAPONS RUSSIA WOULD NOT WANT TO FACE IN A WAR WITH AMERICA - Duration: 14:13.
-------------------------------------------
The Case For Eating Bugs - Duration: 4:29.
Would you eat bugs if it meant helping to save the planet?
I'm Anna and this is Gross Science.
Let me start off by saying that, depending on where you live, the idea of eating insects
may not gross you out at all.
According to a 2013 United Nations report, insects are included in the traditional diets
of at least 2 billion people.
That said, most Westerners, especially in northern latitudes, don't tend to eat bugs—and
in fact, find it pretty disgusting.
So, why is that?
Well, no one knows for sure, but there are a bunch of ideas.
One is that it's simply easier to eat bugs in the tropics, because they tend to be larger,
there's a diversity of species available there all year round, and those bugs may have
predictable harvesting seasons.
Another idea is that traditional agriculture may have made eating insects less desirable.
Agriculture first began in the Fertile Crescent, and then spread to Europe.
And those regions already had large mammals that could be domesticated, so collecting
bugs for food may not have been as much of a priority.
And, as time went on, insects may have gone from being accepted as benign parts of the
environment to being seen as agricultural pests, and invaders in our homes.
Whatever the reason, the fact that most people in the US and Europe regard the idea of eating
bugs with disgust is mostly cultural.
After all, when you think about it, it is a bit strange that our mouths water for this
creature with an exoskeleton and pinching claws and not this one.
Now, you might be saying to yourself, "Who cares!
I still don't wanna have bugs for dinner!"
And to that I say, "Let me lay out the case for entomophagy—the practice of eating insects."
To begin with, insects are efficient at putting on weight.
In order to raise a cow to maturity, you need a lot of food.
But to rear the same amount of cricket meat takes 12 times less feed.
What insects eat is also more sustainable.
We need to devote a lot of agricultural land to traditional livestock—so we can grow
food for them, or so they can graze.
But many bugs can live off of waste products, like manure and compost.
That said, the risks to us of raising edible insects on organic waste are still being studied.
On top of that, livestock like cows are notorious for releasing tons of methane, which is a
potent greenhouse gas.
But very few insects produce methane at all.
Of course, none of that would matter if bugs were bad for you.
But it turns out that they can be a great source of proteins, healthy fats, and minerals,
depending on the species.
Clearly, there are lots of economic, environmental, and health reasons to eat insects—though
we'd have to figure out sustainable ways of harvesting lots of bugs if entomophagy
were to catch on more widely.
But that's not gonna happen if people don't like how they taste.
So, how do bugs taste?
Well, people say crickets taste nutty, scorpions taste like shrimp, and the grubs of the palm
weevil taste sweet when they're raw or like calamari when they're cooked.
And I can tell you that everything I ate today was pretty good, except for those grasshopper
tacos, which were really not great.
But I think that was just probably because I cooked them poorly.
I don't blame the grasshoppers.
I'm gonna put links in the description to all the recipes I used today, and you can
try them out yourself.
I especially recommend the scorpion curry.
And if you do try bugs, let me know what you think, and how you prepared them!
Take a photo of your creations and tag me on Instagram!
I'd love to see what you cook up.
Ew.
By the way, there's one more reason to eat insects—as pest control!
Palm Weevil grubs (the ones that taste sweet or like calamari) are becoming a big problem
in places like southern California.
My friends over at Deep Look actually made a whole video about these guys.
So head over to their channel to see how weevils could harm the iconic palm trees of Hollywood.
And if you like Gross Science, hit subscribe!
That is not delicious.
-------------------------------------------
Video: Muslim teen killed while walking to mosque for prayer - Duration: 1:44.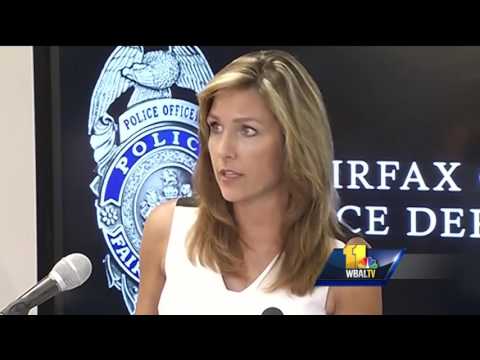
DOWN WITH HIS CAR, AS THEY
DESPERATELY TRIED TO GET AWAY
FROM HIM.
>> WHY YOU KILL MY DAUGHTER, FOR
WHAT?
KAI: THE FATHER OF 17-YEAR-OLD
NABRA HASSANEN.
TRYING TO MAKE SENSE OF WHAT
POLICE ARE CALLING A CASE OF
ROAD RAGE.
THE TEEN AND HER FRIENDS HAD
GONE TO GET FOOD EARLY SUNDAY
MORNING, AND WERE WALKING AND
BIKING BACK TO AN OVERNIGHT
PRAYER EVENT AT A MOSQUE IN
FAIRFAX COUNTY.
WHEN POLICE SAY DARWIN TORRES
FOLLOWED THEM IN HIS CAR, AND
JUMPED A CURB.
>> WHEN I SAW THE DUDE WITH THE
CAR DRIVING ON THE SIDEWALK
THAT'S WHEN I WAS LIKE WHAT'S
GOING ON?
KAI: ACCORDING TO INVESTIGATORS,
TORRES GOT INTO AN ARGUMENT WITH
ONE OF THE BOYS ON A BIKE.
AND ALL OF THE TEENS TOOK OFF
RUNNING.
>> WITNESSES SAY THAT TORRES
CAUGHT UP WITH THE GROUP A SHORT%
TIME LATER IN A NEARBY PARKI
LOT AND GOT OUT OF HIS CAR.
HE WAS ARMED WITH A BASEBALL
BAT, HE THEN BEGAN CHASING THE
GROUP, TORRES WAS ABLE TO CATCH
NABRA.
KAI: POLICE SAY THAT TORRES HIT
NABRA WITH THE BAT.
AND DROVE HER TO A SECOND
LOCATION.
AFTER A SEVERAL HOUR SEARCH,
INVESTIGATORS FOUND HER BODY IN
A NEARBY POND.
WHILE THEY EMPHASIZED THAT THE
INVESTIGATION IS ONGOING, POLICE
SAY, AT THIS POINT, THERE'S NO
INDICATION THAT NABRA'S MURDER
WAS A HATE CRIME.
>> NOTHING INDICATES THAT THIS
WAS MOTIVATED BY RACE OR BY
RELIGION.
KAI: -- IT APPEARS THE SUSPECT
BECAME SO ENRAGED OVER THIS
TRAFFIC ARGUMENT THAT IT
ESCALATED INTO DEADLY VIOLENCE.
KAI: INVESTIGATORS SAY A PATROL
OFFICER WHO SPOTTED TORRES'S
CAR, TOOK HIM INTO CUSTODY A
COUPLE HOURS AFTER THE ATTACK.
HE'S BEING HELD WITHOUT BOND.
LIVE IN THE STUDIO, KAI REED,
-------------------------------------------
Pep Talk | Why Giving is Valuable - Duration: 4:03.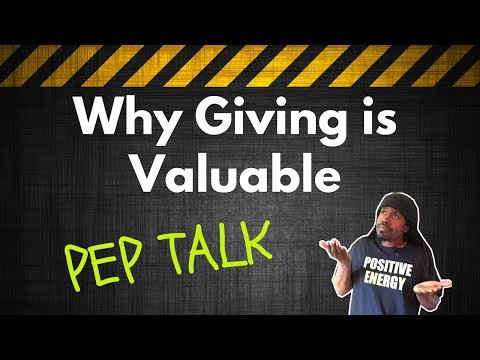
what's up everybody and welcome to another edition of pep talk ride along
so the story goes like this you got two guys mr. a and mr. B now
mr. a is a traveler and he actually you know he's in the wilderness minding
his business going through the mountains and all that stuff
finds a precious stone puts it in his bag right mind in his own business so
here comes along mr. b now mr. B is very hungry he's a traveler
and he sees mr. a mr. a decides to offer him some food
you know so mr. B notices the precious stone and mr. A's bag right
and he's like can I have that right so mr. a without hesitation gives him this
precious stone give it to him so mr. B goes away he's all happy so he
knows that this precious stone is worth a lot of money I mean he's good now so a
few days later the guy yeah I guess he had some soul-searching
you know mr. B talked about mr. B few days later he's looking for mr. a he
finds mr. aids and says here's your stone back he get he returns the
precious stone right so he says to him I want something much greater than this
stone so the guy so mr. B is like I would like to have whatever's inside you
that allows you to give me that precious stone that's pretty deep right
he found that to be more valuable than the precious stone itself the whole
giving so the point of this story is there's a lot more value in giving then
things does that make sense when you're doing your thing you got to think about
it have a lot to offer even if you don't
realize it and this goes beyond just us deep makers in a beatmaker
community it this goes for anything man you you're out and about and you
walk in and you see somebody feeling down if you give them a smile you're
giving them something you know and they will appreciate that so you just get
you're giving yourself you know that's more important than anything you're
giving your time you're giving your energy your positive energy that's why
I'm always on here talking about positive energy man because I'm feeding
off of that because actually when you give you get in return as well you know
I'm saying so the more you give out the more you give back so I feed off of you
guys man that appreciate and write comments and stuff and to be honest with
you I don't even care about these certain videos that don't that they're
not as popular as my other videos because I know that I'm giving and I
know that somebody is still benefiting off of it and it's receiving this you
know because like I say before I'm just reciprocating what I get when someone
gives me I just give it back out so that's all I'm doing so give your time
and give your your energy give that positive energy
give yourself you know because when you give you win so I need to say that again
when you give you win when you hold back and keep everything to yourself you lose
you're losing man give and I need to give this ac some love man because
it's hot so anyway I hope that helps somebody man peace and positive energy
-------------------------------------------
OPV | พูดไม่ได้สักที | 2Moons The Series | พนาวาโย - ก็อตบาส - Duration: 5:20.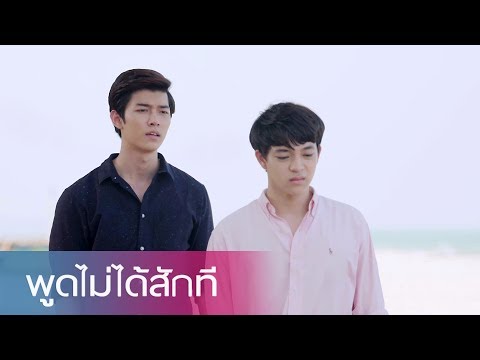
Why are you here?
Nothing.
I just pass by to have a look.
It's pretty far away.
Do you like Yo?
Yes.
His name is Wayo.
We've got the name for you.
You like him, right?
I don't.
I just find it interesting.
Can you just shut up?
I like girls.
What if a boy liked you, you wouldn't have any interest?
Nope.
What happened?
Forth likes you.
So…
I just wanna know what you think about this?
Are you helping him to chase me?
Then, will you say yes?
No!
Yo, what's wrong with you?
Angry?
Yes! I'm so angry!
You never understand my heart!
Do you still remember Nong Yo?
I got his phone number.
You don't want it?
He doesn't like me anymore.
If you don't change your heart, don't you think it's already enough?
-------------------------------------------
Kate Middleton and Prince William News: Is There Truth to the Divorce Rumors About the Royal Couple? - Duration: 2:28.
The Duchess of Cambridge Kate Middleton is easily one of the most envied personalities
in the world.
Her marriage to Prince William of Wales has been the subject of many for years.
They keep a united front and always show a happy family portrait together with their
children Prince George and Princess Charlotte.
But despite all that happy-couple aura the couple exudes, rumors of a divorce still circulates.
Is there a ring of truth to the rumors?
Some publications have reported the Duchess of Cambridge is unhappy with the pressure
the royal family has put on her and feels trapped because of it.
But, a report published by MSN quashed those rumors, with a source stating Kate Middleton
does not feel trapped and is not hiding any marital problems from the public.
Kate Middleton and Prince William recently visited a primary school where Kate addressed
the school full of children on the subject of mental health and illness.
This subject reeks of a sensitive nature and she emphasized the importance of the issue.
She also shared a quote from someone she met that "if you keep your feelings inside, you
can feel as though you will blow up like a balloon.
But, by talking to someone about them, it can make you feel so much better."
Several news agencies have latched onto that and claimed that one of the many reasons why
Kate Middleton was advocating on this topic was because she might be experiencing a similar
situation herself, which, of course, the source close to the situation has quashed and stated
as untrue.
According to an article on Aol.com, the royal couple won't be spending Valentine's Day together
this year due to the couple's separate royal duties.
According to an announcement from Kensington Palace, the duchess will be visiting a Royal
Air Force base in Cambridgeshire by herself on February 14.
This will be her third trip to the base.
She has recently taken over the position as Patron of the RAF in 2015, after the Duke
of Edinburgh served as Air Commodore in Chief for 63 years.
tell us your thoughts in comments below.
thanks for watching.
please like,subscribe and share my videos.
-------------------------------------------
Diffusion-Tensor Imaging of the Physes (July 2017) - Duration: 13:42.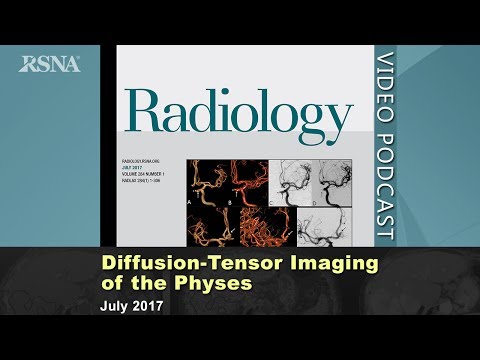
[music]
Ali Guermazi, MD, PhD Good morning. Today we are going to talk about the paper that is going to be in the July issue
of Radiology titled "Diffusion-Tensor Imaging of the Physes: A Possible Biomarker for Skeletal Growth——Experience with 151 Children."
I have the immense, the great pleasure to have the corresponding author with me today,
Professor Diego Jaramillo from the Miami Children's Hospital.
He is an Associate Chair and Professor of Radiology there.
So thank you Diego for being with us this morning and my first question would be can
you explain what prompt you to conduct this study?
Diego Jaramillo, MD, MPH This study is actually the culmination of a number of years of work.
Initially we started working on animal models to look at the tensors in the physes and it
seemed promising so we started taking some – because we were doing some diffusion studies
on regular patients so we would then do the diffusion tensor images and process them in
the physes and we noticed that you could actually look at the columnar architecture of the physes
and the good thing of this is that with conventional MRI you see the physes as a series of layers,
but functionally the physes is really a series of columns and that is what the diffusion
tensor imaging information provides us with.
We thought that we could then start looking at children and we noticed first that, and
this was in a study published in Radiology in 2014, we noticed that the tensors were
longer in the femur than in the tibia and this is what we would have expected if they
would reflect rate of growth because femoral growth is greater than tibial growth, and
then within the femur the growth was greater in the periphery than in the center which
is what we would have expected as well.
That made us think that the modality could be used as a biomarker for growth.
So then we took a cohort of patients that were having MRIs for other reasons.
We excluded any patient who had pathology and then we performed diffusion tensor imaging
on these patients and then recorded several parameters age, gender, BMI, etc. and then
we noticed that there was a striking correlation between growth or expected velocity of growth
in a number of the DTI parameters.
This is actually amazing because you started in 2014 it looks like with animal model and
the discussion of this study that you published and can you just explain in more detail what
the findings are, like briefly and why they are important?
The length has been shown histologically that rate of growth depends on the length of the
columns of converse lengths in the physes.
We noticed that the tensors not only extend into the physes but also into the metaphysis
in the newly formed bone.
But still the column of cartilage in newly formed bone corresponds to the rate of growth.
And so when you start looking at DTI parameters to make the length of the tract or particularly
the volume of the tracks, they correlate well with the expected growth.
So then we looked at the patients and we noticed that in our population the girls started having
longer and longer tracts as they approached puberty and so by age 11 they had a peak and
then the peak came down.
Boys had the same findings but two years later.
These are the same findings that we see for example in the Tanner growth curve where the
greatest speed of growth is in girls around 11 and in boys around 13.
And then we looked at other parameters, for example ADC.
Interestingly ADC does the same thing, but a couple years earlier.
But then we also looked at short patients versus tall patients that were greater than
the 50th percentile.
Of course the tall patients had longer, and this was particularly true in girls, the tall
girls had longer tensors and larger tensor volumes than the short girls.
We haven't done a longitudinal study but it seems to be related to speed of growth.
You said it well.
It looks like this is adding a layer to what the x-ray can inform us about bone growth,
so what is then the real add value of your described method?
The real added value is that you could have a biomarker for growth and have more information
about two things; how much growth there is going to be, and secondly is there physes
getting too close to physeal closure so is there no more residual growth left.
The importance of this is for example with therapies like growth hormone where we administer
growth hormone in patients and it takes about a year or sometimes two years to determine
whether there is response to therapy.
Growth hormone costs approximately $50,000 per year.
If you could identify the subset of patients that are responding to therapy after a few
months, then you could decrease potential complications from therapy and also save a
lot of money.
This is absolutely great idea.
I do think as an application for growth problems is probably one of the most challenging problems
we have today and having a biomarker like this one would be absolutely fantastic.
Then it comes actually to the next question which is obviously if you have this idea about
implementing in treatment in patients with growth problems, do you think this method
can be easily implemented everywhere or do you think the method as any others comes with
some challenges and if there are challenges, can you just tell us about them?
We have used off the shelf products, so this is the diffusion weighted sequence, we used
– all the imaging was done at three tesla on Siemens three tesla units and using the
conventional diffusion sequence that you can get on the magnet with 20 directions and b
values of 0 and 600, so it's something that is readily obtainable really in any of the
MRI units.
Incidentally we did obtain similar measurements at 1.5.
So it's not exclusive to 3 tesla imaging, you can get pretty good imaging at 1.5 as
well and also and then the reconstruction is done with software called Trackvis, but
you can use other software to reconstruct the tensors, but again I mean this is something
that is readily available.
The tricky part is when developing the expertise to sample the right area of the physes, but
this is something that I mean several on our team have become very proficient and it's
– I mean it's not immediately easy, but it's not terribly difficult either.
I mean it's not that more difficult than performing 3D reconstructions for example.
Okay so if I understand you well you use it in specific situation at this point?
Yeah so right now we're conducting a study, an interesting study, with survivors of neuroblastoma,
and these are patients who have a lot of growth problems afterwards and many of them require
growth hormone administration.
Interestingly, we've seen that the for example the fractional anisotropy is very different
for the neuroblastoma patients than for the controls in that the patients who have less
growth have shorter tensors, a more disorganized growth plate and they don't have as a high
NADT so all of these parameters that come from the DTI seems to be correlating well
with the rate of growth in the patients who are receiving growth hormone and that are
having growth problems in the case of neuroblastoma.
The plan, a little bit longer term, is to follow a cohort of patients who are short
and who some of which have growth hormone deficiency and some of which don't, and
also to do a longitudinal study in normal patients because one of the things that we
don't have is longitudinal data.
We don't have – we have cross sectional data from a lot of patients, but we haven't
followed patients let's say for two or three years and that's another thing that we plan
to do.
Very interesting and I'm looking forward to the next data longitudinally.
I hope that you're going to just think about Radiology and to send it there.
Of course.
Looking forward to it.
I have my actually, I do think we are in the last question and I really, I mean I'm actually
have been doing some pediatric radiology in the past, MSK, and to me is actually the bone
age is a big issue that is very important.
So do you think we can use, with confidence, this method in resolving bone age issues or
simply your method just focuses on skeletal maturation?
I think at this point we don't have enough data to have that degree of reliability.
I think one thing that we can predict with a lot of certainty is whether there is growth
potential or not, but beyond that in terms of dating the growth plate or the skeleton,
I don't think we're quite there.
One thing I was going to say is that another application going forward will be for example
in patients who have chronic injuries to their growth plate, the thing that comes to mind
which his very common is patients who have suffered chronic physeal injury due to sports;
gymnasts, baseball players, etc.
Again in these patients it's very important to know, should they stop their activity or
is there a damage to the growth plate or can they continue exercising.
Again, that's another area where we would like to explore.
But in all these cases I don't think we have enough data yet to really know with certainty
for example what is going to be the growth in the next few years and so the only thing
we can say with certainty is there growth potential or not.
Thanks Dr. Jamamillo, thanks Diego.
It's been a pleasure talking to you and thanks very much for joining from Switzerland.
This is tremendous work and it's very exciting and I'm really looking forward to some longitudinal
data that we're going to receive hopefully in Radiology.
Thanks again.
Thank you.
-------------------------------------------
EUROFIRMS | #Bepeoplefirst | Laboral y Prevención - Duration: 2:29.
-------------------------------------------
Mercedes-Benz B-Klasse 180 CDI / AIRCO / AUDIO AF FABR. / LMV / EL. PAKKET / TREKHAAK - Duration: 0:57.
-------------------------------------------
Ford B-MAX 1.0 EcoBoost 100PK Titanium - Duration: 0:57.
-------------------------------------------
BMW 5 Serie Touring 528I High Executive Aut 8 Navi Prof./Xenon/Pano. Dak/Leder/Sportzetels/PDC V+A/B - Duration: 1:01.
-------------------------------------------
Škoda Fabia Combi 1.2-12V Creation - Duration: 0:57.
-------------------------------------------
Diffusion-weighted MR Imaging for Prostate Cancer Detection (July 2017) - Duration: 17:49.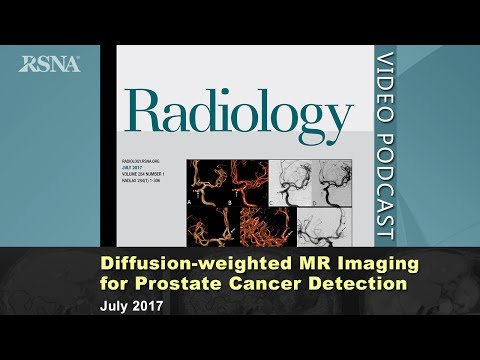
[music]
Herbert Y. Kressel, MD Hi. This is Herb Kressel and welcome to the Radiology podcast.
Today I'm joined by Dr. Andrew Rosenkrantz, Associate Professor of Radiology and Urology
at the New York University School of Medicine.
Dr. Rosenkrantz and his colleagues authored a fairly definitive paper entitled "Diffusion-weighted
MR Imaging for Detection and Assessment of the Aggressiveness of Prostate Cancer: Comparison
between Conventional and Kurtosis Models."
Welcome Dr. Rosenkrantz.
Thanks so much for joining us.
Andrew Rosenkrantz, MD Thanks so much for having me.
Sure.
Many of our readers may only be peripherally aware of kurtosis, diffusion kurtosis imaging,
and its impact in assessing the aggressiveness of prostate cancer in particular, so could
you tell our listeners and viewers what actually is diffusion kurtosis?
Okay so I would compare diffusion kurtosis imaging to conventional diffusion-weighted
imaging which assumes that the water diffusion is free in its behavior.
Diffusion kurtosis imaging recognizes a broader range of hindrances to water diffusion and
can account for those hindrances in its modeling to extract information about the microstructural
complexity of tissue.
It can look at how complex the tissue is at the cellular and even the intracellular level.
So the more complexity of the tissue the less free the diffusion is and that would affect
the kurtosis.
It also affects the distribution of the diffusion parameters.
Correct.
Okay.
With that as a basis, why would you think this kind of a metric might be of value in
assessing the aggressiveness of prostate cancer in particular?
So for assessing the aggressiveness, prostate cancer we're really talking about the Gleason
score which currently it's a score that the pathologist determines on their visual
assessment looking at the tissue under the microscope.
That is really driven by the architecture, the complexity of the tissue in its appearance.
The conventional ADC is kind of more of this hodge- podge or amalgamation of facts also
influenced by tissue viscosity, other factors, and the hypothesis is that the diffusion kurtosis
is more of a kind of a pure or less contaminated reflection of really just the structural complexity
and hindrances which its believed could more reflect what pathologists are trying to estimate
when they assign the Gleason categories.
Thank you.
Now this study is a follow-up to your own which we published in 2012 was a preliminary
assessment of diffusion kurtosis imaging in prostate cancer, and since that one was published
there have been several others published and the results as you note have been mixed.
Why did you think that this current study you're reporting could more definitively
determine the utility of kurtosis in looking at the aggressiveness of prostate cancer?
Were there particular problems with the prior studies?
Yeah, so the studies I've been – so this has been a topic of a lot of interest in the
last few years, we're all trying to best predict the Gleason score non-invasively which
has huge implications for prognosis and treatment selection and the studies have had mixed results.
Some have been really promising, others have not been.
So in terms of some of the limitations of what's been done is the sample sizes have
generally been small.
There were some use of the reference standard biopsy instead of radical prostatectomy as
we did in our initial feasibility study of kurtosis in the prostate which has diffusing
biopsy has limitations in terms of the precision of the grading, and even just some technical
factors in terms of how the kurtosis image sets are processed and the metrics are obtained.
I think some of our understandings of this have evolved and improved over the last few
years, and I think in terms of what b values from a diffusion acquisition are incorporated
and how this is done.
It's not just pushing a button.
There's a lot of optimization of the (inaudible.)I think even that has gotten more precise as
well.
Good.
Can you briefly outline what you actually did in your study?
Tell us a little bit about the design and sort of what you looked at.
We had a sample, a retrospective sample, of several hundred patients.
At our institution our standard diffusion acquisition provides the necessary information
to be able to extract the kurtosis metrics.
All the patients underwent prostatectomy after MRI.
We included patients where pathology had done detailed mapping of the distribution of tumor
within the prostate including localization and grading of individual tumor foci.
We then selected the dominant or index tumor in each patient, and correlated these back
to the imaging and placed regions of interest to obtain what we deemed to be the optimal
ADC, standard ADC, and the optimal kurtosis metric that we could generate using our acquisitions.
Were these mean ADC and mean kurtosis over the whole volume?
It was the mean value of an ROI on the higher lesion.
And then we correlated that back to the pathology and we looked at the ability of ADC and kurtosis
to separate benign versus malignant as well as to separate low grade from high grade tumors
using two different definitions of high grade that have been applied in the literature.
So really we had three different histologic outcomes for which we compared conventional
ADC and kurtosis.
Okay and what were your key findings?
In terms of our findings, we found these interesting.
ADC and kurtosis they were highly correlated.
As ADC goes down, kurtosis goes up.
Both of those show as being generally associated with greater aggressiveness.
Their accuracy for predicting our various end points, benign versus malignant or high
grade tumor, were very similar.
When we looked at the subset of patients let's say there was a difference between them, kurtosis
actually did not outperform ADC within that subset.
So either they generally gave – for most patients they predicted the same thing.
Either ADC and a kurtosis said could be benign or be high grade or both of them said there'd
be, they both gave similar results or basically giving – to say it somebody's giving some
redundant or repetitive information from the kurtosis that we already have from ADC and
in the small minority when they were discrepant, the ADC actually did similar or even a little
better.
Although in the paper you describe what I would consider a fairly rigorous protocol
for matching the images particularly the target lesion with histopathology.
In 36 patients the target lesion couldn't actually be identified on the MR images.
Were you surprised by this and why do you think it might have happened?
So that's a really important point.
Those were overwhelmingly low grade tumors.
These are Gleason 6 lesions.
This is recognized actually in prostate cancer, this is a recurring issue whenever you try
to do detailed MRI pathology correlation for prostate cancer.
And other groups have described, other (inaudible) have described this as well.
Some prostate tumors they don't really form a discrete mass like say a renal cell carcinoma,
it's just it's histological sparse.
The malignant glands are interspersed with benign glands.
There's really no actual discrete tumor and these can actually just be invisible on
imaging.
For those we had no clear correlate on the imaging.
And for those in our final analysis, we ended up basically looking at the numbers both ways
where we just empirically placed an ROI and where is seemed the lesion was based on pathology
versus just excluding those.
Whether we did an empiric ROI or we left those out we had similar.
Our final observations held true.
Okay.
Now although the ADC and the kurtosis findings were correlated and concordant and around
80%, as you noted they were discordant at around 20% and you mention that in these the
majority of them the ADC was sort of more accurate or at least correlated better with
the histopathology, but my question is what do think is driving the discordance and what
sorts of lesions did you notice this discordance?
ADC and kurtosis they were correlated but these aren't – there are differences.
The ADC does have influences from viscosity concentration.
There are some other – it is kind of more this kind of this messier metric whereas the
kurtosis is really more tuned into complexity and hindrances to water motion.
There will be a small fraction where they didn't exactly follow each other.
I can't say exactly what the unifying kind of property was of the lesions where that
happened, but that it was not common and the ADC was either similar or a little better
than kurtosis in that.
So what you're saying is that they are in fact, although they're related, they're
measuring somewhat different things and it's not surprising that the measurements wouldn't
be the same in some subset of patients.
Exactly.
Now as we said in your study you looked at whole ROI or most of the lesion volume ADC
and kurtosis.
Others have started to look at subsets doing histograms of the ADC and the kurtosis looking
at sort of the worst actors, the bottom 10%, bottom 25% and have you looked at other kurtosis
based metrics?
So I think the histogram metrics for extracting a summary value from diffusion acquisitions
is really promising.
So say looking at the 10th percentile or (inaudible) this has shown I mean some really compelling
results in the prostate as well as in a range of other organs where work from other centers
or work that we've done at NYU.
I think unlike the diffusion kurtosis technique this doesn't really require any changes
or adjustment in the protocol or a standard protocol can be done and it's all on the
post processing side.
I think vendors are making those tools available and this is I think becoming easier to incorporate
into clinical workflows.
So I think this has a lot of potential in the near term.
The same thing with the kurtosis method or a histogram of it?
And this can be completely applied to the kurtosis data set which we didn't do in
this study, but I mean it's a great idea.
The literature has consistently shown incremental improvements in performance when applying
the histogram analysis.
So whether done to a conventional ADC, you could do a conventional diffusion analysis,
it could be applied in a comparable way to the kurtosis data set.
It wouldn't surprise me if that led as well to incremental improvement there.
In this study we were just doing a comparison of conventional mean between kurtosis and
standard diffusion, but I think I would anticipate that applying a histogram approach to either
of these could bump up their performance a bit.
Yeah I mean histologically as you know the Gleason grade can vary throughout a lesion
in scattered areas and so it would seem that sort of you know the and my understanding
is that the worst grade drives the final grade so that this might more closely mirror what
the pathologist is looking for and sort of finding the most aggressive areas to establish
the grade.
I agree with you, it sort of makes sense as an area for future investigation.
This sort of leads up to my final set of questions for you, it sounds like you haven't given
up on kurtosis yet.
Are you still acquiring this data in your patients?
We're looking at, I think more can be done to optimize it and there might be some additional
ways of, some additional angles that haven't really been as well explored or considered.
So no we haven't given up on it.
I think it can be something that can be combined with other innovations.
As an example, more recently what we're turning our attention to is time dependent
diffusion.
This is a parameter that hasn't gotten quite as much attention by radiology investigators.
A lot of the focus has been on the b value and directions of the underlying diffusion
acquisitions.
There's also the actual diffusion time.
The time between the diffusion (inaudible) gradients actually can be manipulated.
It's playing with the actual source sequence.
The vendors don't always make as readily available to us as radiologists to work with,
but it can be, it actually can be adjusted and we're coming back and actually – we
can actually push it to really short diffusion times, shorter than are usually applied and
can technically we tune the contrast of the diffusion image sets in that manner, we can
actually go back and combine that with the kurtosis analysis or the conventional diffusion
analysis; and then we're finding some really interesting results that way.
So we're still using kurtosis but the idea is to combine this with some other manipulations
of the diffusion acquisition and we'll see what it shows over time.
Sounds like there may be another Radiology podcast in your future as you get more into
this work.
Dr. Rosenkrantz it's been a pleasure chatting with you about this paper, and I think at
this point in time it certainly gives us a good handle on the relative role of diffusion
kurtosis imaging in looking at prostate cancer aggressiveness.
Thanks so much for joining us.
Thanks so much again and I really enjoyed participating.
Great, bye-bye.
Bye.
-------------------------------------------
Pias Tip für Forever Aloe Gelly - Forever Aloe Vera Gelly - Duration: 1:12.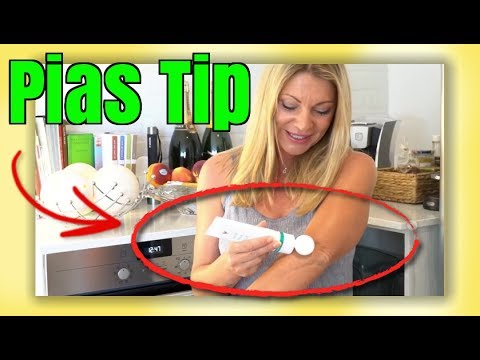
-------------------------------------------
Fruits and Vegetables | Coloring Pages Fruit names Kids Learning | How to Draw Fruit and colors kids - Duration: 13:49.
-------------------------------------------
Análise Jogo Internacional x Paraná | Prognósticos de Futebol Brasileiro 2017 Série B - Duration: 3:21.
-------------------------------------------
Brève de brasseur : Edward, brasserie de l'Être - Duration: 2:07.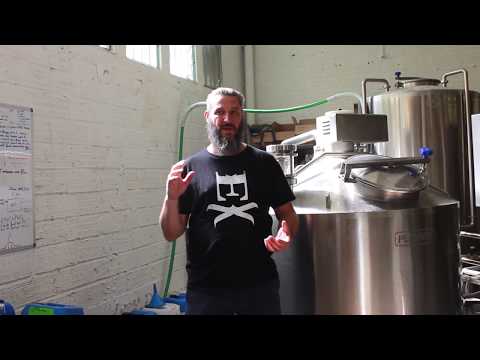
-------------------------------------------
How to Draw Chicken, Coloring Pages Animals for Kids | Art Colors for Children with Colorful Markers - Duration: 7:22.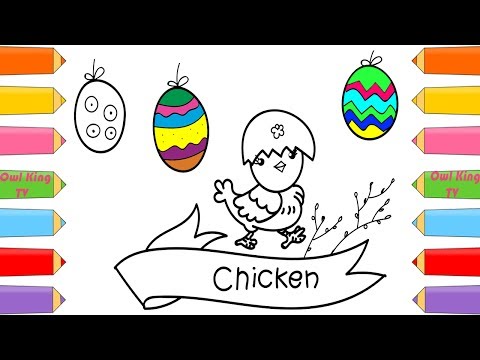
How to Draw Chicken, Coloring Pages Animals for Kids | Art Colors for Children with Colorful Markers
-------------------------------------------
La famille pour tous - Grandir avec un parent handicapé - Duration: 0:31.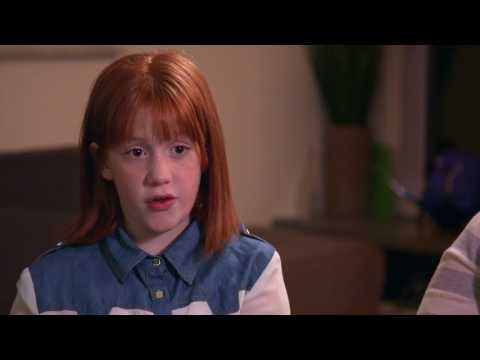
-------------------------------------------
For more infomation >> La famille pour tous - Grandir avec un parent handicapé - Duration: 0:31.-------------------------------------------
Autour du travail : Annonce Interview #3, Charline, infirmière - [Engl. Subs.] - Duration: 2:33.
Serie : Behind the work
Visible Human collective
Are you ready for a new interactive experience ?
What if we travelled a bit more together...
In search of 'the Other' ?
Between the familiar and the unfamiliar...
Your own stories are welcome !
Charline, a nurse who graduated in 2010, has held her own private practice since 2013 and is on her way to providing home care.
To avoid professional isolation and fulfill the need to "speak to someone", she began writing to clear her mind and share with her entourage.
The following year, after taking her friends' advice and without great conviction, she launched a blog titled : "It's the Nurse!".
Today, it contains over 200 articles, with over 1 million visits…
What does the nursing profession mean to you?
Let's plan this interview together…
Let's meet on Facebook or YouTube
Until the 25th of june, you can leave your questions in the comments section or on our Facebook page !
Since we'd like all of you to understand and share this french project (and since our English is perfectible... !) We're looking for some people who could kindly help us to translate our interviews and reports ! THANK YOU !!
-------------------------------------------
For more infomation >> Autour du travail : Annonce Interview #3, Charline, infirmière - [Engl. Subs.] - Duration: 2:33.-------------------------------------------
I'm With Ameya - Duration: 1:47.
I'm with Ameya because he's not a part of the political machine.
Because he'll stand up to billionaires and special interests who put profits over people.
I'm with Ameya because he understands the intersections of race and class, in a way
that reflects itself in its criminal justice reform, rural and education policies.
Because he stands with the LGBTQ+ community as we fight for dignity, equality, and justice.
Because he's not a Springfield insider or a career politician.
Because he believes in the future of our state, not just the past.
He's a fellow first generation American.
He gets that whether black, white, or brown, we all just want a fighting chance at a better future.
I'm with Ameya because he has real solutions for the problems we face, not just more tired
rhetoric.
Because Illinois deserves a budget for mental health care.
Because he believes women's rights are human rights.
I'm with Ameya.
I'm with Ameya.
I'm with Ameya.
Everywhere I go I hear the same thing.
People are sick and tired of the millionaires and billionaires and insiders getting ahead
and a majority of working families get left behind.
It's time for a progressive change.
It's time to forge a New Deal for the State of Illinois.
When I ran for office in 2011, I didn't have any money.
I didn't have any endorsements and the insiders all laughed at me.
So I got to work.
I knocked on every single door.
I listened.
I talked to people.
And I brought my community together.
That's how I beat the Chicago machine.
This is how we're going to beat Bruce Rauner.
And so today, I'm asking why you're with me.
Tell me your story.
Use the hashtag #ImWithAmeya and let's go out and forge a new deal.
Thank you.
-------------------------------------------
BMW 3 Serie Touring 330D M-PAKKET PANO-DAK - Duration: 0:56.
-------------------------------------------
Finding Dory Remix - Duration: 2:32.
Finding Dory Remix
I'm Dory Dory, help me find my family
I'm Dory can you please help me?
You are coming to the ocean with me Hi I'm Dory
Dory, help me find my family I'm Dory can you please help me?
You are coming to the ocean with me
**Autotuned fish**
I suffer from short term memory loss short term memory loss
short term memory loss short term memory loss
**Screaming fish** **Dolphin Jam**
Remember, Remember What were we talking about
Remember, Remember I think I'm going to remember you
Remember, Remember That's the one thing I can remember yeah
Remember, Remember Have you lost your marbles
Oh Oh Oh Oy Oh Oh Oh Oh Go
Oh Oh Oh Oy Oh Oh Oh **bird screech
**Autotuned Dory** Finding Dory Remix
**Screaming fish** **Dolphin Jam**
I'm Dory Dory, help me find my family
I'm Dory can you please help me?
You are coming to the ocean with me
Hi I'm Dory
Finding Dory Remix
-------------------------------------------
Renault Scénic 1.6-16V Expression Sport *APK T/M 5-2018* (Airco/ECC/Trekhaak/LMV) - Duration: 1:01.
-------------------------------------------
Fiat Punto Evo 1.3 M-Jet Edizione Cool 5DRS - Duration: 0:54.
-------------------------------------------
How to Become Rich - Duration: 3:51.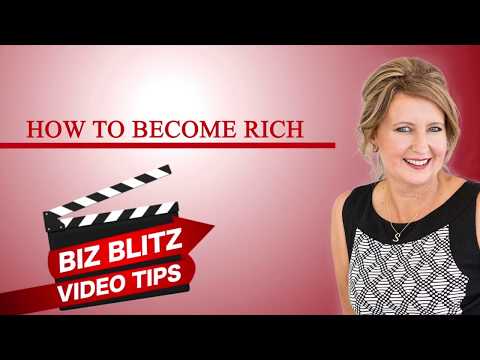
Hi I'm Sharon Jurd and welcome to my Biz Blitz video series.
And today I want to talk about the three do's and don'ts of building a website.
Now I'm not going to get into IT heavy stuff, I'm not an IT guy, I don't look like one,
I'm not one, never have been one.
What I want to talk to you about is coming from a layman's term,
a business owner who doesn't do IT and I want to talk to you about that today.
The first thing I want to talk to you about is when you start to build a website allow extra time.
If you think you want a website in X amount of weeks,
double it because once you get going you'll actually get excited and go,
"Oh and I want this and I want that and I want this and can we do that?"
If you're a creative person particularly you'll keep adding on all the time.
When people say to me, "Oh, I have a website up in a month." I go,
"Oh you're kidding me because when you say a month you mean two. If you say a week you mean two."
Get the time frame to be realistic before you even start.
Give yourself plenty of time and don't be stressed over a certain deadline.
When you're thinking about doing that start very early in the pace.
The other thing is don't make it difficult for your customers when they arrive on the website.
I see so many websites where there's a one hundred and one things on there;
there are so much content, there are so many photos and graphics,
there are so many tabs, it just becomes overwhelming. And people won't go there if it's not a user-friendly site.
It has got to be clean, it has got to be crisp, it has enough information on there to get them excited,
wanting to contact you and then you can talk to them further.
But if you have everything you do and everything about what you do.
The customers are just going to be overwhelmed and a confuse customer never buys.
They have to have clarity around your message and why they need to engage you or at least contact you.
The third thing is, go live! It won't be perfect straight away,
make changes as you go along because if you wait for your website to be
"perfect"
then it will never ever go live and I've heard of people spending twelve,
eighteen months saying I've got to make a few more changes.
That's ridiculous! Just go live and say, "Okay it's 70, 80, 90% there."
Your customers are probably not going to notice unless there's a link broken.
If your links are fine, a little bit of graphic, a little bit of content or changing a photo here and there,
they're not going to notice so much that's just your insecurities about perfection.
So go live, make changes later and you'll probably get some comments
from people about this and that so you can make the changes when they comment.
So they are my three points: allow extra time, don't get stressed over a tight deadline.
Two, don't make it difficult for your visitors coming there and finding information about you.
And three, go live! Make changes later.
Your website will be never ending of changes. It will be a continuance throughout your business life.
Now in 12 WBA, my 12 Week Business Academy online program,
we go into this in more in-depth.
I actually go through the questions you need to ask your website builder and your website designer.
And what the difference of those are to know IT jargon, it is basic business owner content.
I don't get in to the nitty-gritty of algorithms.
I don't even know how to spell it, I have difficulty saying it, don't need to know it.
That's what your website builder needs to know but you need to know the questions to ask him
and that's what I cover off more extensively in my 12 Week Business Academy.
If you want to know more go to www.12wba.com.au
and watch my very short video there and I'll tell you all about what's in the program
and I hope that I see you in my 12 Week Business Academy.
I'm Sharon Jurd.
Thank you for your time today and if you think that this video will add value to your friends, your family,
your colleagues please send it out to them.
Share, share, share, share, share because I would love to share this video to as many people as I can.
And with your help, I know that I can reach more and more people.
So thank you in advance for sharing my video today.
I'm Sharon Jurd and I will talk to you very, very soon.
-------------------------------------------
[Japanese ASMR] Marshmallow Eating Sounds #2 / Whispering - Duration: 15:01.
-------------------------------------------
Things That Make Me Happy - Spring 2017 | @laurenfairwx - Duration: 6:51.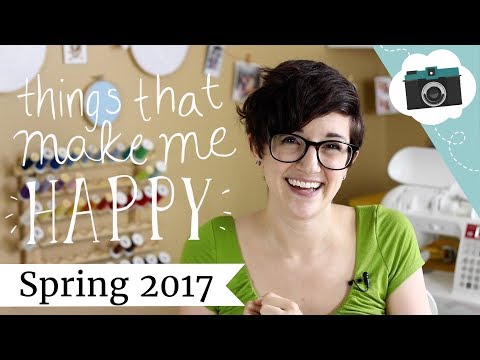
Just under the wire, I wanted to do a spring edition of my Things That Make Me
Happy series. I think of this as a more conceptual kind of favorites video
because some of my choices are pretty general. But honestly, the things that you
don't have to go out and buy are sometimes just as happy-making as the
products that I really like. I might as well just get started, so here is another
list of things that make me happy! First up for this episode: Easter candy. The
best time of year for chocolate, in my opinion. Russell Stover milk chocolate
covered marshmallows and the Cadbury caramel eggs are some of my absolute
favorites. But for some reason the Reese's Peanut Butter Cup eggs taste so
much better to me than just normal Reese's peanut butter cups that you can
get all year long. I don't know why that is. Maybe because it's fresher and it can
only sit on the shelf for a limited amount of time before it becomes
outdated and it goes to clearance, which, clearance Easter candy is also something
that makes me really happy. After Easter is over, they mark it all down and it's
still just as delicious, so I just go and buy a whole bunch of it and then try to
make it last as long as I can. I know it's a silly thing, but I get so excited
for Easter time because, I just, the chocolate makes me really happy.
I don't even wait until Easter to eat it. I start eating it after Valentine's Day
when it appears in the stores magically and it's just the best thing. The next
thing on my list is long walks. It sounds so simple but after being
cooped up all winter long, trying to walk in the snow and people just don't shovel
their sidewalks in my neighborhood, it is so nice to go outside, sometimes without
a coat, and just enjoy the fresh air and I love it. There are nice things to look
at, I sometimes run into friends in my neighborhood and get to catch up, and it
makes me feel a lot better. If I feel like I've been cooped up all day and
just like sitting and working for hours, it's so nice to go out for a walk. It
inspires me to do work, so when I come home, I have like a ton of new ideas to
work on. It's something that I've found is really good for my mental health. I
love it. I love going for walks! The next thing that makes me happy is Julie's
Greenroom. This is Julie Andrews' Netflix original show and it
just is so cute! I was so excited when I heard that the show was going to happen
because it's like everything that I wanted in a show when I was a kid. I have
a one and a half year old. Getting to enjoy it with him, and I'm sure I'm going
to watch it again when he's a little bit older and can really understand all of the
concepts, but it's a show about theater and the arts! It's Julie Andrews teaching
a new generation of kids about musicals and it's so positive and sweet. There's a
really great special guest on every episode. I really needed this show right
now. It's just, it makes me really really happy and I highly recommend it if you
haven't watched it, especially if you're a theatre person. So the next item on my
list is Japanese stationery. Ever since I got into traveler's notebooks, I've been
looking online at all sorts of cute stickers and pens and I feel like I've
been gravitating the most towards things that come from Japan. I've been a little
bit overwhelmed with all of the options, so I haven't bought very much Japanese
stationery, but then Zenpop, which is a stationary subscription service located
in Japan, offered to send me a box of their stuff so I said yes. Knowing me, you
may be able to imagine the restraint and the patience that it required for me to
not open this until I got on camera, but I'm going to check it out now. Oh it's
really cute. There's actually a lot of stuff in here!
Stickers and a little memo pad, oh my goodness this is cute.
I don't know what it is about this stuff. It like, makes me really excited. Some
really adorable pencils. Oh my gosh, washitape with cats on it. Little sushi
stickers! Anyway, that gave me a really good place to start with my Japanese
stationery addiction, so thank you, Zenpop. I think I'm in trouble
because I'm going to go buy a whole bunch more now. The next thing is Roller
Coaster Tycoon Classic for the iPad. Mobile games are great, I really like
them, I play a whole lot of them, but any time I play any mobile game that's at
all theme park related, I keep thinking back to when I was in middle school and
I would play Rollercoaster Tycoon, the original version of it, and I kept
thinking, how do all these mobile games that have all this new technology at
their disposal, not coming even close to at least what I remembered of the
Rollercoaster Tycoon of my childhood? So the people who made Rollercoaster Tycoon
decided to re-release the original version of
Rollercoaster Tycoon but reformatted for the iPad. I was so excited when I found
this out. It really is just as good as I remember, I don't know if the children of
today would feel the same way, because I'm sure it's a nostalgia element. I
think it is so much fun to play. It's such a silly, fun game but it's a huge
game! It's massive! There are so many parks. It includes some of the original
expansion packs as well. If you ever played that game as a child, you have to
look it up. I cannot recommend it highly enough. And the last thing on my list of
things that make me happy for spring, is Instagram! Now, I've been on Instagram for
a long time. I really enjoy it. It's an extra creative outlet for me and I love
the idea that I can take a photo on my phone and then mess with it in a bunch
of different apps for a while, and then post it and share it with people
immediately and I just think that's really fun! Another thing that I love
about Instagram is that it is what you make it, so if you want to follow a ton
of artists that inspire you, all you have to do is open up Instagram and just
scroll through your feed and when I do that I get inspired, I get excited to
make things again. It sometimes can turn my whole mood around and just make my
day a little bit happier and that's kind of amazing. People are always quick to be
negative about social media and there are a lot of negative things about it.
But I find that Instagram is one you can use it in this specific way, to only
follow things that make you smile and that can be kind of powerful, you know?
I've also been posting more often on Instagram lately because it's been
making me so happy, so if you want to follow me on Instagram and see what I've
been posting, I've got a whole bunch of behind the scenes stuff for my channel
and my crafts and my shows that I perform at! Look me up on Instagram at
@laurenfairwx. Okay! So that's it for now. What kinds of things have been
making you happy lately? Tell me in a comment below and hopefully I'll have a
chance to reply if I can. I love chatting with all of you in the comments. If
you're new here and you liked this, don't forget to subscribe and hit the
notification bell so you don't miss any of my future videos. I've been working on
a lot of happy and creative stuff lately and I can't wait to share it with you.
Thank you for watching and I'll see you soon!
-------------------------------------------
Audi A1 Sportback 1.0 TFSI SPORT 95pk Xenon, 16"LM - Duration: 0:59.
-------------------------------------------
Swimming in Laguna 69 at 5° - Huaraz Peru - Duration: 1:34.
5°
He went in
Have you ever seen a crazy person go in when it is this cold
When it is this cold no
Crazy
Crazy people
Never before?
Crazy American
Not that cold
People swim when it's warm but...
Perfect
-------------------------------------------
Happiness Is A Process Not An Achievement - Duration: 5:17.
How's it going everyone I'm dono and this is how to happy.
In this video we're going to be talking about being happier instead of trying to be happy.
So you may have heard the phrase I'll be happy as soon as this thing happens or I'll be happy
when la de da de da.
Then that makes happiness come across as some kind of binary state right we're either happy
or we're unhappy and for a lot of people we're looking for this future event or some kind
of thing and we say to ourselves I'll be happy when I reach this milestone or whatever it
is.
But the problem is you reach that milestone or whatever you're looking forward to and
yes you are happy for a while or what you feel is happy for a while but then it kind
of wears off and you're looking for the next milestone to hit and then go back into - ok
I'll be happy as soon as I do this, because a lot of times the milestone isn't necessarily
as different as expected to be because in our day to day experiences we're not taking
the time or we don't have the tools to be happy.
So instead of thinking about happiness in terms of just one destination it's a lot more
helpful to think about it as an ongoing process and going along with that instead of being
a destination and with it being a process it's something that you work at a little bit
every day.
Now this does a couple things for you.
1.
It's going to make being a little bit happier and happiness more approachable.
When you see and think about every single day okay what can I do today that will make
me a little happier.
What can I do tomorrow that will make me a little happier.
You don't have this really strong burden of the binary on-off happiness type switch which
doesn't really exist, right.
Happiness is more along a spectrum it's not that you're happy or unhappy there's differing
degrees of emotionality that are connected to happiness.
In addition when we look at happiness as a process it's something that we can continually
work on right.
Even when you get into those on states of happiness you're probably not actually optimal
right if you're thinking about it as a binary switch but when you're thinking about it as
a process where you work on being a little happier each day then you can continue working
at these things.
That's going to take away kind of that feeling of oh I'm unhappy today what's wrong with
me why aren't things going well.
No.
It's because it's not a binary switch, it's a continuum so life ebbs and flows there's
going to be days where we're happy or unhappy but hopefully over time the graph looks something
like this and it's trending upward as we learn more tools and more ways to control our environment
and to influence our happiness.
Now another thing that switching your thinking from a destination to a process is going to
do is that when make mistakes or when you're having a bad day you're not going to be looking
at happiness in a binary way and think oh man I'm not happy today so I must have failed.
When you reach those milestones and they're not what you think and you get disappointed
again then you're not going to beat yourself up even more because you can look at it and
say okay what thing can I do today to be a little happier and just keep working on that
way.
That way you're not going to ever run into these really big gaps where you, you know
you've gotten everything you've ever wanted or everything you thought you wanted and you
still don't feel happy.
So in summary, thinking that we can just be in this constant on state of happiness doesn't
really make sense, it's not really a place that we get to and achieve, there's too many
things that happen in like and cause bumps and change things up.
So if I can get anything across and I've probably repeated a thousand times already in this
short video but if you can just switch from thinking about happiness as a destination
to a process where every day you look for things that will make you a little happier
and guide you toward more happiness that's going to be a much more realistic and helpful
framework that allows you to continue working on your happiness regardless of what happens.
Then as you build up these habits over time you're going to find yourself getting happier
and happier and being able to deal with some of the drops a little bit better.
That being said let's move on to the exercise section.
What is one thing you can change in your life today to be a little happier?
So that's all I've got, like I said try to find one thing that you can do today that's
going to make you a little happier whether it's a little happier today or a little happier
tomorrow.
Just one little thing that you can work on that's actionable that can get you started
in the right direction.
As always if you have any questions, comments, topics you want me to cover put them down
in the box below and I will get to them as soon as I can.
And I will see you next time.
You can check out more content at www.howtohappy.com also don't forget to subscribe so you can
stay up to date on the videos.
We've also got a twitter facebook and instagram you can check out.
Reflect, take action, and enjoy life.
-------------------------------------------
RMS Nomadic & Pigeon Pirates | We See Here - Duration: 1:39.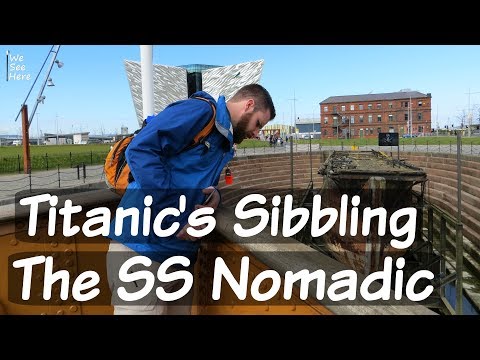
We See Here
We See Here
We See Here
We See Here
The Great Wilderness
We see here, as we stand upon,
the HMS Nomadic.
The ship that was once a sibling of the Titanic.
Which as you can see behind me,
is the very famous Belfast Titanic Museum.
One of the best museums that you could ever go to.
And yes, it does have a ride inside that is enjoyable.
Um, but if you come over this way
Over here to the edge,
and look down.
You can see that we are in a dry dock.
And over there is the never before sailed US...
aaaaa, EUS Pigeon.
A pirate ship built entirely by pigeons
It was never sea worthy.
Hence it is still in the dry dock.
Catch us next time as we find historic boats that never sank,
while we talk about boats that did sink behind us.
Ah...Yes.
Không có nhận xét nào:
Đăng nhận xét