None of my friends will talk to me now including Varun
Why are you crying?
I have got dandruff
Apply curd
All the dandruff will go away
The dandruff is gone
The curd is also not going from my hair. This remedy is not working at all, dandruff is still there
Why are you crying?
I have dandruff
You want to get rid of dandruff?
Apply this egg on your hair
Don't eat it
The dandruff is gone
Due to this egg, my hair is spoilt but the dandruff is still there
Why are you crying?
This dandruff has ruined my life
Apply this beer
If drinking beer was a remedy to remove dandruff it should have gone a long time ago
Huh?
I never did it
Apply only on your hair
The dandruff is gone
Did dandruff go?
No, dad. I won't go out from now onwards
None of the remedies worked
Before I only had dandruff, now it's become more worse
Sometimes I feel, I have washed my hair with vomit
Nothing has worked
Why are you crying?
This dandruff is not going at all
You have dandruff? Do you know why you get dandruff?
It happens cause of Malassezia
One second
I have tried many shampoos. It doesn't go
This is the new head & shoulders shampoo + conditioner
It has zpt which clears all the germs of dandruff
The conditioner will keep your hair smooth and silky
OMG, the dandruff is gone
You looking so beautiful
I shouldn't have wasted the beer
Your looking nice
What is there in your face?
A pimple?
What?
I have a remedy
You leave it. I have a better remedy
Apply toothpaste
Oh My!
Let me know in the comment section, which home remedies actually works
What ever is the remedies let me know
If you do everything, all would be alright
For more infomation >> Home Remedies: Expectation VS. Reality | Rickshawali - Duration: 6:43.-------------------------------------------
Full BCA file released in Thurman Blevins shooting - Duration: 2:40.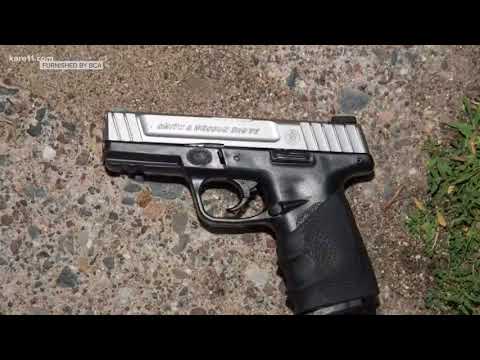
-------------------------------------------
Chaos After School Bus Drives Wrong Way On One-Way Street In Brooklyn - Duration: 1:52.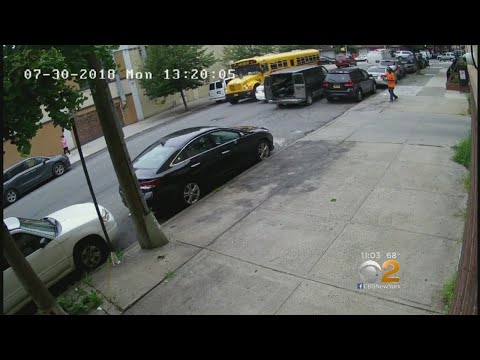
-------------------------------------------
Noticias Telemundo, 30 de julio de 2018 | Noticiero | Telemundo - Duration: 22:21.
-------------------------------------------
Video Of Alleged Arsonist On Staten Island - Duration: 0:26.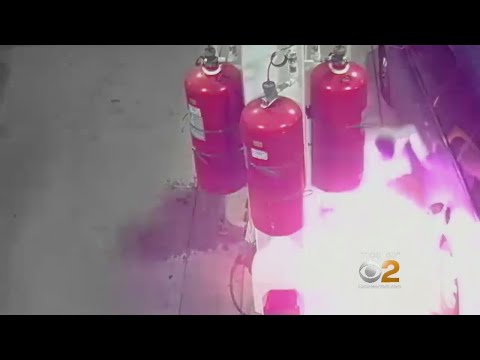
-------------------------------------------
Fight between Astros fans and homeless man - Duration: 1:46.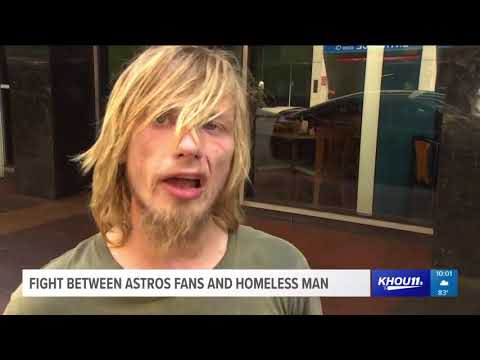
-------------------------------------------
Driver involved in head-on crash that killed new father was out on bail - Duration: 1:45.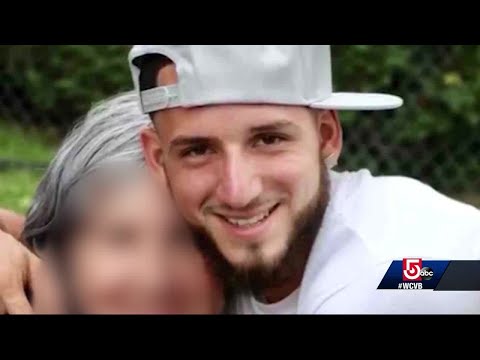
-------------------------------------------
Shark stolen from San Antonio Aquarium is returned home - Duration: 2:56.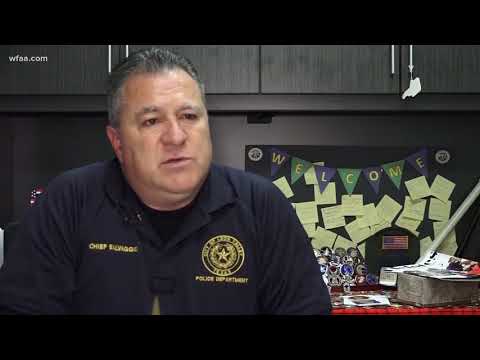
-------------------------------------------
Police say 'Insurance Burglar' caught in the act - Duration: 2:01.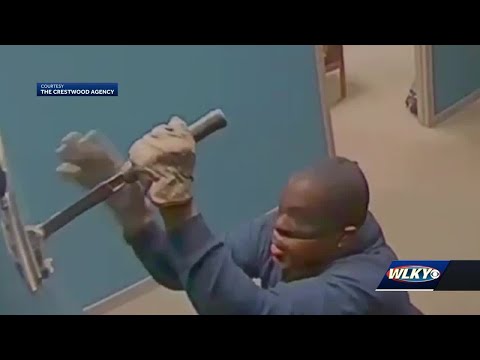
-------------------------------------------
No charges against Mpls. officers in Blevins shooting - Duration: 1:50.
-------------------------------------------
Mother, baby rescued from floodwaters - Duration: 1:47.
-------------------------------------------
Tackling a huge banana split at Training Camp - Duration: 2:12.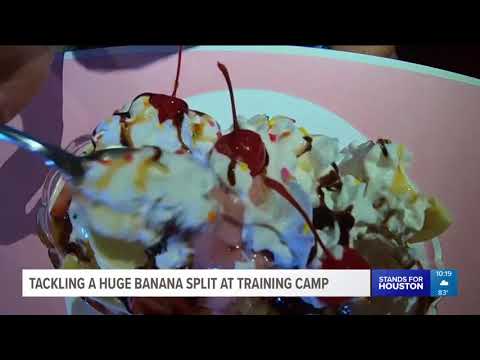
-------------------------------------------
'I'm not used to this.' Red Sox fans snarled by bridge project traffic - Duration: 1:21.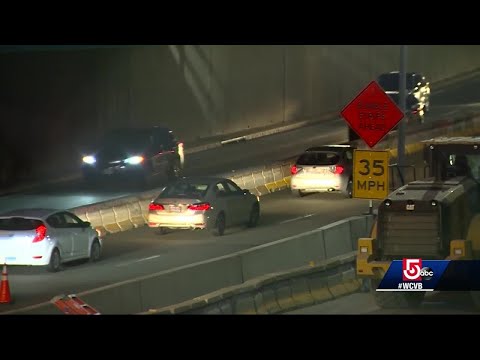
-------------------------------------------
Big bucks spent on city, county car washes - Duration: 3:20.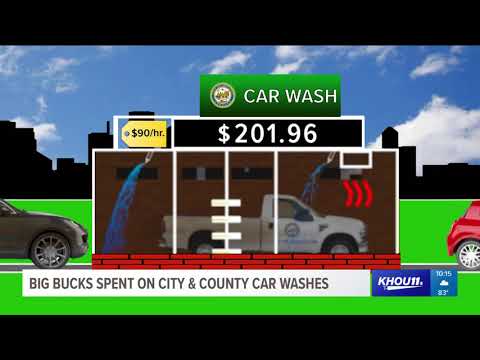
-------------------------------------------
Council Bluffs community mourns loss of longtime athletic trainer - Duration: 1:58.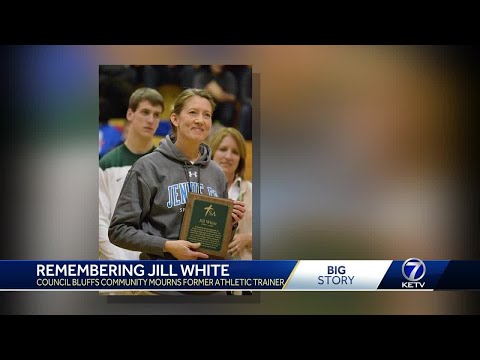
-------------------------------------------
Small plane removed from Connecticut River near Haverhill - Duration: 0:34.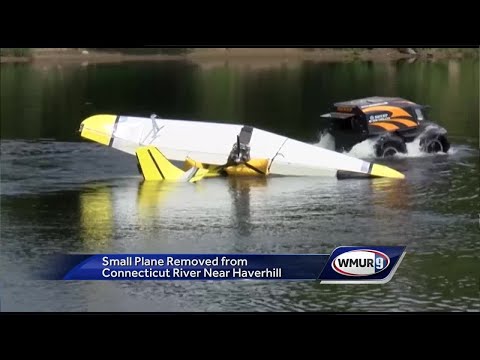
-------------------------------------------
Newmarket man accused of posing as DEA agent - Duration: 1:43.
-------------------------------------------
Lecciones que aprendí de Joven (Lessons I Learned as a Boy ~ Pdte. Gordon B. Hinckley) - Duration: 4:04.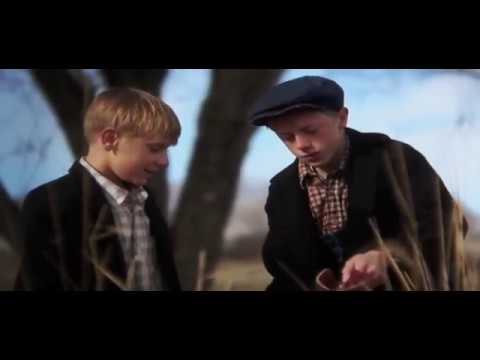
-------------------------------------------
Chapter 9 Lecture 3 - Duration: 9:02.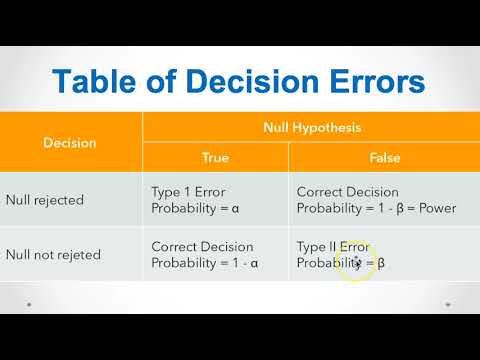
Hello everyone.
This is our 3rd and last lecture for Chapter 9 – An Introduction to Hypothesis Testing
In this lecture video, we will discuss decision errors and power.
Because the hypothesis test relies on sample data, and because sample data are not completely
reliable, there is always the risk that misleading data will cause the hypothesis test to reach
a wrong conclusion.
Hypothesis testing is an inferential process.
It uses limited information to reach general conclusions.
Sample data are used to draw conclusions about a population.
There is always some probability of error in the results.
Two types of errors are possible: Type I errors and Type II errors.
Type I error is the probability of rejecting the null hypothesis when it is, in fact, true.
Type I errors occur when the sample data appear to show an effect when, in fact, there is
no effect or difference.
In this case, the researcher will reject the null hypothesis and falsely conclude that
there is a difference or effect.
The researcher rejects a null hypothesis that is actually true.
Type I errors are caused by unusual, or unrepresentative samples.
Just, by chance, the researcher selects an extreme sample with the result that the sample
falls in the rejection region even though there was no actual effect or difference.
The probability of making a Type I error is equal to alpha, α.
Type I errors are also known as "false positives."
Examples of Type I errors happen in the medical world when someone gets the results from their
biopsies and they are told they have cancer (but they really do not have cancer).
Or drug testing…someone fails their drug test when, in fact, they should have passed
it (because they are drug-free).
Someone is convicted of a crime they never committed.
Type II error is the probability of not rejecting the null when it is, in fact, false.
Type II errors occur when the sample does not appear to show an effect or difference
when, in fact, there is a true effect or difference.
In this case, the researcher will fail to reject the null hypothesis and falsely conclude
that there is no difference or effect.
The hypothesis test failed to detect a real effect or difference.
Type II errors are commonly the result of a very small effect.
Even though there really is a difference, it is not large enough to show up in a research
study.
There is an inverse relationship between Type I and Type II errors.
The probability of Type II errors increase as the probability of Type I errors decrease.
The probability of a Type II error (NOT Type I) equals beta, β.
Type II errors are also known as "false negatives."
Examples of Type II errors happen in the medical world too: someone goes to the hospital and
they are sent home, when they should have been admitted to the hospital (the test did
not find the "bigger" problem).
Or lie detector testing…someone passes the lie detector test when, in fact, they should
have failed it (because they are lying through their teeth).
Someone is acquitted for a crime they actually committed.
Here is the table of decision errors or what we fondly refer to as "The Confusion Matrix,"
because it tends to confuse students.
This table shows that there are four decisions we could make: 2 decisions are errors (Type
I error & Type II error) and 2 of them are correct decisions.
Power, 1 - beta, β or 1 - alpha, α (not alpha it's Type I error); 1 – alpha is
the correct decision.
What we will be focusing on next is Power, which is the probability of correctly rejecting
a false null hypothesis.
Power is the probability of detecting an effect that truly exists.
Power is equal to not making a Type II error (NOT Type I) or 1 - beta, β.
There are a number of factors that affect power, or the probability of correctly rejecting
the null hypothesis.
So let's take a look at what factors increase power:
Increasing sample size, n, decreases standard error, increases test statistic, which increases
likelihood of rejecting the null, which will increase power and vice versa (decreasing
sample size, decreases the likelihood of rejecting the null, which will decrease power)
Increasing alpha, α, increases the rejection area, increases likelihood of rejecting the
null, which will increase power and vice versa Increasing mean differences between M and
μ, increases our test statistic, increases likelihood of rejecting the null, which will
increase power and vice versa Increasing effect size, increases power and
vice versa Decreasing population standard deviation,
σ, decreases standard error, which increases our test statistic, increases likelihood of
rejecting the null, which will increase power and vice versa
Conducting a one-tailed or directional hypothesis test, increases the rejection area, which
increases likelihood of rejecting the null, which will increase power and vice versa (a
two-tailed test decreases our rejection area, decreases likelihood of rejecting the null,
which will decrease power)
This leads us into the factors that affect hypothesis testing, which are related to power:
Increasing sample size, n, decreases standard error, increases the test statistic, which
increases likelihood of rejecting the null (and vice versa)
Increasing mean differences between M and μ, increases test statistic, which increases
likelihood of rejecting the null (and vice versa)
Decreasing standard deviation, σ, decreases standard error, increases test statistic,
which increases likelihood of rejecting the null ( and vice versa)
One last thing we need to discuss is the assumptions for hypothesis testing.
In order to conduct what we call parametric hypothesis tests, we must meet three assumptions:
1.
Random sampling – we assume that data were obtained from a random sample
2.
Independence – we assum that each outcome or observation is independent, one outcome
or observation has no effect on another 3.
Normality – we assume that data in the population are normally distributed, especially for samples
of n < 30.
In larger samples of n > 30, this assumption is not as critical (remember due to the central
limit theorem).
This concludes our coverage for Chapter 9.
I will post one more video that will show you how to conduct hypothesis tests, both
directional and non-directional.
This video will also show you how to write appropriate APA conclusions.
-------------------------------------------
心理測試:你的拇指握拳伸直是哪種?秒測你天生屬於什麼命相? - Duration: 4:37.
-------------------------------------------
McMinnville trio returns to 'America's Got Talent' for 'Judge Cuts' episode - Duration: 1:52.
-------------------------------------------
林志玲穿紫裙高貴,周冬雨穿紫裙清純,劉濤穿紫色裙好冷酷! - Duration: 3:18.
-------------------------------------------
Giovanni Bomoll Music
-------------------------------------------
'I'm not used to this.' Red Sox fans snarled by bridge project traffic - Duration: 1:21.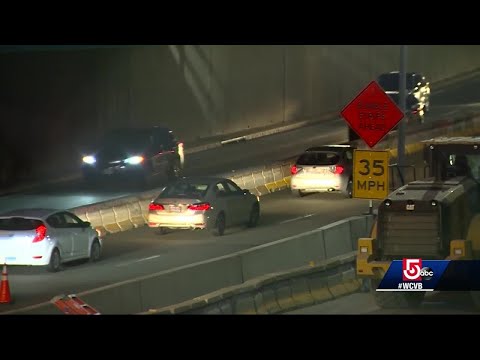
-------------------------------------------
Southern Charm Savannah: Hannah Breaks Down At Dinner With Ex Louis (Season 2, Episode 3) | Bravo - Duration: 1:26.
-------------------------------------------
The Wolf Of Wall Street I'm Not Leaving - Duration: 0:52.
-------------------------------------------
Classic Smokey Eye Using Addiction Palette 3 - Duration: 6:46.
Hey girls, okay. Today's video is going to be all about this look right here. It's gonna be a classic
Smokey eye but I'm gonna break it down and make it a little bit more
Simple for you, so it's like a beginners smokey eye, but I think it's still really beautiful. It's a classic
That's Addy in the bathroom potty-training right now
It's a classic look. I used one palette a couple brushes
and that's it so if you want to see how I achieved this look then keep on watching and
Hopefully it will be helpful
Hey girls, okay. So as always we're gonna start with primer
I'm pushing the primer into my eyelids using a sponge primer is
Such an important step
Then I'm going to take some translucent setting powder and stamp out an angled line from the outer corner leading up to my brow
This is going to help give me a visual for how I want the eyeshadow to be shaped
It's gonna make sure I don't go outside that line
Next I'm gonna go in with a pale gray shade using a fluffy blending brush
I'm gonna start at the outer corner and start buffing this into the crease
I'm using really tiny short little motions to go in a back-and-forth windshield wiper
Motion this is gonna start to build up our crease and give us this shape
You don't ever want to go in with your darkest color
you need to slowly build it up a
Grey is a great color to start it smokey eye with as I get most of the color in that outer corner
I'll start to take it over to the inner corner, but you always want to be starting at that outer corner
Next I'm gonna mix two shades the same light gray that we went in at first with a little bit of a darker one
Using the exact same brush. I'm gonna buff it into that outer crease. So it's almost like I'm drawing a sideways V
That's how you create that smoky cat eye shape
You'll notice that a lot of this look is just me blending going back forth and blending in small circles
It's such an important step. Okay. Next we're going to go in with the darkest colors is a pure black
I'm taking it little brush and I'm drawing a sideways V
I'm literally just stamping that color into the outer corner. It doesn't mean to look perfect
It doesn't need to look pretty because now what we're gonna do is blend blend blend this color
so you can even take a black eyeliner and push it into the outer corner of your eye and
Blend it to get a smoky eye that works just as well
I think a powder is a little bit easier to work with but that's just me
So again, I'm stamping it in that outer corner and then going back with a fluffy brush and blending blending blending
You're always blending towards the outer corner, but notice I'm not taking the color all the way towards the inner inner corner
I'm leaving that
Pretty light and bright that's how you can avoid getting really dark heavy eyes that can make your eyes look smaller and tired
Keep all that darkness towards the outer corner
Okay, next we're gonna take a small liner brush I'm gonna spritz it with a little bit of setting spray
Then I'm gonna go back with that dark black color and I'm gonna line underneath my eyes with it
I'm keeping it as close as I can to the lash line and this is where it's okay if it gets a little bit
Messy or smudged out. That's kind of the point. It's to look a little bit messy and undone
so push it in there and just
Smudge and blend it out
I sprayed it with setting spray to make sure that it's really gonna be locked on there and no raccoon eyes then
I'm just taking a little bit more and darkening up that outer corner a little bit more. You can totally play with an intensity and
Say hello to the two-year-old
Okay now I'm gonna go
eyeliner
I'm going to tightline my upper lid so I'm pulling it. I'm putting the child down to force just weren't doing it
I'm pulling my lid up and pushing that liner into the upper waterline
This is gonna make your eyes look dark in smokey Nicks and help your lashes to look thicker and more
Balumptuous' and then I'm gonna line the lower waterline as well
I only do this when I'm doing a really dark intense, or I look normally I wouldn't line my eyes with a black eyeliner
But for a smoky eye, it's a definite then I'm taking the liner and pushing it into my upper lash line as well
Okay, now I'm gonna take my finger and that sounds crazy spritz it with some setting spray
I'm gonna take a light shimmery shade and Pat this into the eyelid
I have found that a lot of times with shimmer shades
Your fingers are the best thing you can use
If you don't use your finger, you're gonna want to use a flat synthetic brush, but your finger works great
you can really pack on the color and I sprayed my finger with the setting spray to reduce any fallout and make sure that the
shimmer, really pops
Okay next I'm going to go back into the black shade and just add a little bit more there in the outer corner sometimes when
You add on the shimmer the black can get a little bit diluted
So I just want to make sure that it's really dark and pigmented
I'm gonna go back in with that shimmer shade and taking a tiny little brush
I'm gonna use it to highlight the inner
corner of my eye and my brow bone this helps to bring together the whole look and the last step is to
take off that powder first of all, but then to add on a ton of
Mascara the more mascara the better
You could even pop it on a pair of false eyelashes
If you want to I'm using the epic mascara
Which is my favorite and I feel like it gives you just as much length and volume as false lashes do so for me
That's good
I also I'm going to take it on to my lower lashes
Which is something I don't normally do but with a smoky eye you want
Lashes all around so go ahead and put it on those bottom
bottom baby lashes
And that is it girls, that is a smokey eye for you hope you enjoyed
-------------------------------------------
2⃣0⃣0⃣जवालामुखि योग का अनुभव पार्ट-66||nirvachan problem solution||brahma kumaris meditation experie - Duration: 1:22.
-------------------------------------------
Book Attack | #BookTubeAThon 2018 [CC] - Duration: 1:52.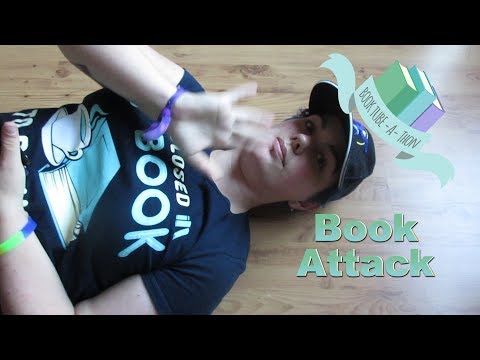
["Funin and Sunin" plays throughout]
-------------------------------------------
Melbourne footballer suffers footy banner fail - Duration: 1:41.
Melbourne footballer Tegan Young played game 100 for the Seaford Tigerettes on the weekend, and as is the tradition, a banner was made for Tegan to run through pre-match
However, the banner had other ideas, and the video of Tegan's attempt has now attracted a lot of upvotes on Reddit, with users weighing in on the funny video
"Congratulations Tegan on 100 games!! still 99 games!! - wordtwoyamum"Dusty would be proud
" - Zenderbobo"This is my favourite thing of the week. Early call, I know, but I'm confident
Gold!!" - RjCharlie"Classic stitch-up." - drunkillAt least the club can be proud there were no spelling mistakes, a problem that has caught a couple AFL cheersquads on the hop this year
Watch the video in the player above to see what all the fuss is about.
Không có nhận xét nào:
Đăng nhận xét